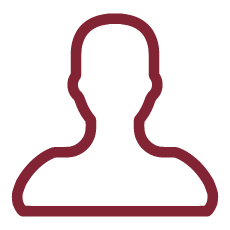
Background: In recent years Artificial intelligence (AI) has represented a very innovative research field with applications in several of medical branches, in particular in medical imaging. In fact, AI is particularly prone to be applied in medical imaging due to the large amount of data generated through image acquisitions available in digital format, that enable the AI process of ¿learning¿ from huge dataset. Among AI methods, deep learning (DL) has been recently implemented for Magnetic Resonance Imaging (MRI) protocols applied on different anatomical regions with interesting feed-backs in terms of image quality and reduced acquisition time. Up to now, a few study assessed the potential benefit of DL applied on upper-abdomen MRI in clinical routine.
Aims:
1. To evaluate image quality of the deep learning-based upper abdomen MRI protocol compared to standard non-deep learning protocol.
2. To compare acquisition time between deep learning-based MRI protocol and standard non-deep learning protocol.
Methods: Thirty patients who will undergo upper abdomen MRI we will be prospectively included. Each patient will perform a dedicated MRI protocol with the same scanner (1.5T GE Signa Voyager, GE Healthcare, Waukesha, WI) including axial T2 single-shot fast spin echo (SSFSE) sequences and axial Diffusion Weighted Imaging (DWI) acquired with deep learning (DL) method (AIR¿ Recon DL, GE Healthcare, Waukesha, WI) and non-DL with standard protocol. Acquisition time will be recorded for each sequence. Each acquisition will be anonymized and two readers independently will assess image quality by quantifying signal-to-noise ratio (SNR) and contrast-to-noise ratio (CNR) drawing circular region of interest in the liver parenchyma, in the image background and in the gall-bladder. Moreover, visual image quality including sharpness, contrast, artifacts and overall quality will be performed by using a five-point Likert scale.
AI has represented a very innovative research field with applications in several of medical branches, in particular in medical imaging, where advantages has been enormous an still in progress. In fact, AI is particularly prone to be applied in medical imaging due to the large amount of data generated through image acquisitions available in digital format, that enable the AI process of ¿learning¿ from huge dataset.
AI has multitude applications in different aspects of medical imaging work-flow. In fact, AI is applicable in many fields including a) image acquisition and reconstruction, b) image post-processing, c) image analysis and visualization, d) image storage, e) integration with medical records and f) integration of complex data for medical decision making process.
Among different imaging modalities, Magnetic resonance imaging (MRI) is one of the most recent techniques introduced in 80s and it represented a cornerstone in medical imaging due to the lack of ionizing radiation and the possibility to provide brilliant soft tissue contrast and multiparametric information. Despite incredible advantages MRI suffers from long time of image acquisition and the constant technical difficulties related to image setting due to several parameters to balance. During these decades, several improvements has been performed to reduce scanning time preserving image quality such as techniques that allows the partial acquisition of k-space or new sequences able to reduce motion artefacts. However, to find the perfect balance between image quality and acquisition time is still challenging and compromise often is the best possibility due to physics limits hard to overcome. In this contest AI represents a powerful method able to optimize the image acquisition process. In particular, deep learning (DL) and machine learning (ML) approaches have been tested on MRI acquisition with excellent results. An example in provided by AIR¿ Recon DL - ARDL- (GE Healthcare, Waukesha, WI) method applied on MRI. ARDL is a new method able to elaborate from raw k-space data (input) high fidelity images (output) reducing image noise, truncation artifacts and improving edge sharpness. The innovative approach proposed allows to integrate the pipeline directly during the acquisition process on k-space, not as superimposed post-processing filter. In fact, ARDL technology is based on a convolutional neural network (CNN) containing over than 4 millions of near-perfect high resolution training data that allow to reduce truncation artifacts and noise also with acquisition parameters set to reduce scan time (i.e. reduced matrix, reduced averages). By applying ARDL, images obtained have the potential to preserved incredible image quality in with reduced acquisition time. Up to know, a few studies have assessed ARDL protocol in clinical routine setting and image comparative studies are needed to assess the potential benefit of this new technique. In fact, AI may consistently improve MRI technique in terms of diagnostic confidence obtained with better image quality and in terms of improved clinical work-flow due to reduced acquisition time.