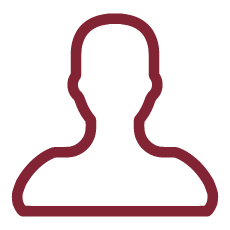
In the last decades the climate change and other complex systems presented new challanges to forecast extreme natural events, these need new stochastic models and statistic tools in order to be modelled and simulated. Furthermore, many complex systems show the property of long-memory: the past times have a not negligible effect on the current time. Some examples can be provided by earthquakes, stock markets and Pm pollution.
The fractional processes in time or in space are able to describe such extreme events thanks to their densities' fat tails but the way in which the long-memory can be defined is still an open problem.
Indeed, mathematically, these characteristics can be expressed by the long-range dependence and the selfsimilarity property: the long range dependence expresses the correlation of the values the system takes in two distant times whereas the selfsimilarity explains the dynamic itself of the system for two distant time. Specifically, each property can be summarize by one parameter provided in each definition.
In order to make these definitions useful for applied sciences, estimators will be defined so that scientists can apply these statistical tools to get the parameters which describe long range dependence and selfsimilarity.
The aim of this research is to define a new theoretical framework where the selfsimilarity and long range dependence could be studied. Indeed, the approach of renormalization group and phase transition started to be used by Samorodnitsky but it is not yet performed for fractional point processes.
Using our approach, the definition of selfsimilarity and long range dependence can be applied to fractional point processes, using the well-known theory of CTRW.
The definitions of selfsimilarity and long range dependene will be linked strongly by working on the space {P(u), u is in U} where statistic considerations can be done easier than on the process itself.
We will be able to enlight the relation between the fractional parameters and long range dependence, so that not only the fractional process can be applied more easily but we will identify a statistic tool able to quantify the strongness of dependence in data sample coming from counting processes.