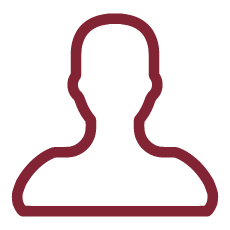
Quantum technologies are expected to seriously affect various scientific and daily aspects of modern society. Relevant examples are the simulation of quantum systems, materials engineering, nano-technologies and internet commerce. Machine Learning - how a computer can learn from data - is a lively research area that has advanced quickly over the past few years: its applications are ubiquitous and range from e-commerce, healthcare, to neuroimaging, particle physics, fundamental science.
The aim of MAC QUANTUM is to experimentally address the connection between quantum information and machine learning. By exploiting a hybrid integrated photonic platform, we provide an experimental breakthrough in this research area, considering both "hardware" and "software" contributions, and pursuing three objectives:
1. To develop novel hybrid integrated quantum optical circuits. The goal is to increase the effective Hilbert space dimension, which can be efficiently manipulated for information processing purposes.
2. To demonstrate that integrated photonics can be fruitfully adopted to implement quantum machine learning. We will carry out a variety of on-chip demonstrations of quantum machine learning by exploiting quantum interference/entanglement as building blocks to manipulate vector states in large dimension Hilbert space.
3. To exploit machine learning for new insights in quantum physics. On this purpose MAC QUANTUM will extend our capability to engineer quantum states, unitary evolutions and generalized quantum measurements. We will develop an approach able to self-design the manipulation of quantum states depending on the desired task.
This innovative project goes far beyond the state of the art and promises to capture truly new scientific horizons at the frontier of quantum information, quantum physics, quantum control, machine learning and integrated photonics.
A central question in the philosophy of science regards the existence of fundamental limits to our capacity of learning about the world. For half a century, information theory and theoretical computer science have been delivering both fundamental and practical answers about inference and learnability. This has helped shape our modern, information-based economy.
Machine learning (ML) is the field of computer science that utilizes algorithms to learn and to make predications from input data: its applications are ubiquitous and range from e-commerce and healthcare, to neuroimaging, particle physics and fundamental science.
The relatively recent emergence of quantum information (which combines computer science and quantum physics) grants major implications for ML . In fact, such promising implications have led scientists and corporations like Google and Microsoft to target their research efforts on the performance of ML and other computational tasks through quantum computing. The reason for this is simple - classical computation is limited in terms of processing speed, and quantum computation can solve certain problems exponentially faster than with any existing computer. This is getting more and more important in the modern era due to two paradigms: 1) The explosion of data ("Big Data") originating from research and real-world applications, for instance in biological data, financial markets, weather forecasting, audio/video processing, as well as from exponentially growing and interconnected intelligent sensor networks such as the Internet of things; 2) in about 2020 electronic chip size will shrink down to the atomic level where quantum mechanics applies: accordingly Moore's law is expected to be not more valid.
Recent developments in quantum information have raised the prospect that near term quantum devices can expediently solve computationally intractable problems in simulation, optimization and machine learning. The results of this research have led to a newly emerging interdisciplinary research area between quantum physics and computer science called Quantum Machine Learning (QML). QML can provide efficient solutions to quantum information having vast implications for machine intelligence while introducing a new approach to the elaboration of information within network infrastructures. This is an ambitious challenge with a very large potential impact.
Quantum Information (QI) implementation on a chip is expected to open an era of revolution in integrated photonics technology. The realization of complex optical schemes consisting of a large number of elements that can be interconnected to exploit the main features of quantum phenomena of increasing complexity requires the introduction of sophisticated on-chip technologies. Hence, photonics quantum technologies may have a significant real-world impact: this scenario represents an opportunity to develop further these technologies thereby leading to important spillovers.
The aim of this project is to experimentally address at a foundational level the connection between quantum information and machine learning by exploiting an hybrid integrated photonics platform. The approach is to set up a photonics-driven approach to QML with both "hardware" and "software" contributions.
We will develop the underlying technology and carry out several experiments in order to highlight the potential for machine-learning primitives to obtain enhanced performances in quantum information processing. We will utilize statistical learning theory to address fundamental quantum problems, such as the inference of unknown quantum states or operations, or the hidden structure of the underlying quantum system. As following step we will address the experimental foundation of quantum machine learning.