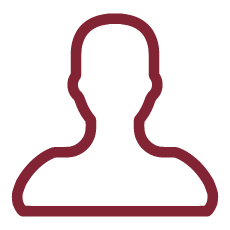
Despite the simplicity of its molecule, water displays the most complex phase diagram of any single component system, characterized by multiple stable and metastable phases, and with new phases still being discovered. Both the thermodynamic and dynamic properties of water are often at odds with the behaviour of simple liquids. These "water anomalies" have their origin in an experimentally inaccessible region of the phase diagram known as no-man's land, where computer simulations hint at the presence of an elusive liquid-liquid phase separation. Close to this region, water displays both arrested (glassy) and crystalline phases. The characterisation of these phases and of the processes through which they are connected requires a microscopic understanding of the structure of water molecules under extreme conditions. Traditional descriptors of local order, also known as order parameters, are low-dimensional representations of the local environment around a water molecule. While conceptually simple, they are known to have poor spatial resolution, and have lead to misidentifications problems in a large number of previous studies.
In this project we plan to fundamentally change the way water local structures are studied. We abandon the idea of low-dimensional order parameters, and instead describe water with high-dimensional quantities, where information about the real space structure is retained as much as possible. In order to manipulate these quantities we then employ Machine Learning methods, such as supervised and unsupervised Neural Networks.
These new high-dimensional descriptors of water's local environment will then be applied to study the process of ice nucleation under supercooled conditions. We plan to extend our Machine Learning techniques to also characterize the non-equilibrium glassy states of water.
For countless natural and biological processes, and for a vast array of industrial applications, water is one of the most important substances on Earth. At the same time, it is also one of the least understood. Understanding its thermodynamic and dynamic behaviour is thus of paramount importance.
The main difficulty we face when studying water's phase behaviour is the fact that many of water's phase transitions, such as freezing, originate from fluctuations that are very localized in space and time. For this reason, computer simulations, which make it possible to keep track of the degrees of freedom of each molecule, are giving a fundamental contribution to the understanding of the phase behaviour of water. However, up to now the order parameters developed to characterise the local environment of water, and hence of the many phase transitions that characterise its behaviour, suffer from two drawbacks: they have a low dimensionality and they are lossy. In this project we plan to overcome both limitations by using high-throughput Machine-Learning-inspired algorithms. Indeed, the Neural Network approach not only will improve our resolution and precision, but holds the potential for new avenues of investigation, for example quantifying the role of metastable phases in nucleation (Ostwald step rule). Research in this direction has been very limited [13], and so far nobody has attempted to enhance the existing order parameters with Neural Networks, which we believe makes this research very timely.
REFERENCES (see also "Descrizione obiettivi progetto" and "Inquadramento della ricerca"):
[13] P. Geiger, and C. Dellago, J. Chem. Phys. 139, 164105 (2013)