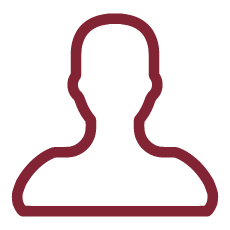
The aim of this project is to develop a new approach based on partial differential equations for mixture models in the field of soft clustering problems. In particular, our purpose is to conceive a procedure, based on the solution of a multi population Mean Field Games (MFG in short) system, which consists of coupled Hamilton Jacobi Bellman and Fokker Planck equations, to compute finite mixture of Gaussian and Bernoulli distributions. These models are important tools in the statistical analysis of data, exploited to find the presence of subpopulations (clusters, groups) within a overall population. In the classical setting, the Expectation Maximization algorithm is performed in order to compute the optimal parameters of the mixture. The novelty of our method, based on MFG theory, relies on the infinite dimensional optimization aspect that can be employed to discover new properties on cluster analysis and can be generalized in several directions. An interesting aspect, in the particular case of isotropic Gaussian mixture, is the convergence of the corresponding MFG system to a coupled partial differential equations for hard clustering problems. Finally, we are interested to extend this approach to the evolutionary setting, in order to model the time evolution of clusters and analyse polarization effects.
This project will bring a new perspective for a class of problems which, up to now, have been considered in the classical framework of finite dimensional optimization. This is a first step toward the application of partial differential equations theory in cluster analysis. The new approach will allow to use the theory of PDEs to discover new properties and structures of clusters and will provide a more flexible model that can be adapted in different situations.