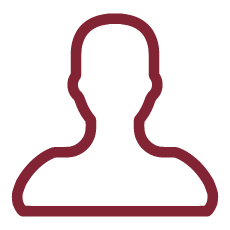
The modern revolution in information technology, which has been significantly impacted by the advent of Internet-of-Things, has allowed us to interact in advanced ways with a plethora of mobile devices, technological artefacts embedded in the surrounding environment, and entire systems. It is hard to deny that the "user interaction everywhere" phenomenon has changed our way of living and relating. The general feeling is that interactivity is a positive feature, associated with being flexible, informed, and in control.
Among the most relevant aspects of a user interaction, this research projects focuses on the concept of learnability, which applies to the nature of the performance change of a user when interacting with a system to accomplish a specific task. The interest in defining and measuring learnability of interactive systems is acknowledged by the presence of several research studies that are available (from more than four decades) in the Human-Computer Interaction literature. Nevertheless, to date, there is no a shared understanding of the concept of learnability and, as a consequence, several accepted metrics exist to assess the initial learnability of a system, which represents (at best) a measure of the system's intuitiveness and is not necessarily an indicator of continued learning.
This research project starts from the idea that measuring the degree of learnability of a system over time (the extended learnability) is crucial for understanding exactly what happens during user interactions and for designing transparent, explainable and interpretable interactive systems. To tackle this challenge, this project investigates how a targeted use of techniques and algorithms from the Process Mining community provides a natural framework for: (i) discovering interaction models that precisely describe user interactions; (ii) measuring if an interactive system is learnable with respect to its tasks, by detecting potential learning issues and ways to fix them.
This research project is timely and potentially ground-breaking in the field of HCI, as nowadays there is an increasing number of software applications that offer task-based interactions through mobile devices or (directly) via the surrounding technological environment and use to record such interactions in dedicated logs and send such logs back to the developers of the software, who must then make sense of them [17,18].
Our research project moves towards the realization of an approach aimed at measuring the extended learnability of an interactive system. Among the quantification of learnability, the grand challenge of this research is to understand exactly what happen during user interactions in order to design interactive systems that will be more and more transparent, interpretable and explainable. This challenge is also in line with the recent trends in HCI of investigating the human behaviours during an interaction for detecting, for example, potential security leaks that affect interactive systems [19].
Our approach aims at advancing the state-of-the-art in HCI by tackling this major challenge following three innovative and unexplored research directions in the HCI field.
First, the approach is thought to be employed during the daily use of a system without the need of observing directly the interaction of the user with the system, an aspect that makes it completely different from the traditional learnability approaches, which always require a direct user involvement in controlled (lab) experiments and, consequently, are suitable for measuring "just" the initial learnability of a system.
In addition, explicitly recording user interactions in dedicated logs allows checking the degree of transparency of such interactions, which can be dirty by the presence of user actions that are expected to happen in the interaction models but are never really performed by the user (e.g., because they are not visible in the user interface).
Second, the approach enables to automatically quantify the extended learnability of an interactive system, which has proven to be complex and costly to be calculated. Specifically, it allows evaluating the learnability of a relevant task of the system on the basis of the level of user's experience (i.e., distinguishing between novice and experienced users) by precisely detecting potential learning issues and their severity.
All this aspects guarantee the user interaction to be explainable. This means that the approach is able to produce user-oriented explanations of how the objective of an interaction is reached, identifying which user actions have been responsible to cause learning issues and suggesting reparative actions to be incorporated into the interaction model for solving the learning issues.
Third, the approach allows the automated discovery of human-computer dialogs (i.e., interaction models) starting from the interaction logs. To date, interaction models are only seldom used in HCI to model very specific features of an interaction, since asking designers to define interaction models "by hand" at the outset is hard and not appropriate.
Our approach aims at automatically extracting interaction models in form of Petri Nets starting from the interaction logs. While it is undoubtable that there are aspects of the interaction that can not be formalized through Petri nets, including the objects manipulated by user actions and the representation of the users roles (that can be modelled by richer languages, e.g., the standard language ConcurTaskTrees [20]), the advantages of Petri Nets is that they provide a formal semantics, which allows to interpret the meaning of an interaction model unambiguously, with the possibility to perform on this models any kind of automated reasoning.
References:
[17] A. Holzinger et al. What do we need to build explainable AI systems for the medical domain? Tech. Report. arXiv:1712.09923 (2017)
[18] F. Cossu et al. Improving operational support in hospital wards through vocal interfaces and process-awareness. In CBMS, IEEE (2012)
[19] J.D. Still. Cybersecurity needs you! Interactions, vol. 23, n. 3. ACM (2016)
[20] G. Mori, F. Paternò, C. Santoro. CTTE: Support for Developing and Analyzing Task Models for Interactive System Design. IEEE Trans. Software Eng., vol. 28, n. 8 (2002)