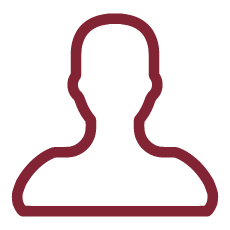
There exists a strong relationship between volatility and stock market performance; volatility tends to decline as the stock market rises and increase when the stock market falls. Volatility has a lot of applications for investing, trading and predicting of direction of the stock market.
Recently the VIX, Volatility Index, also labelled as the Fear Index, traded on the Chicago Board Options Exchange, has become the most leading indicator of stock market trends and a hint of what is about to come in the global economy.
Generally, movements in the stock market can be quite volatile and they sometimes seem separated from economic factors. However, there are some underlying factors which have a strong influence on the stock prices movements and the stock market in general.
In my research, I am working on the realistic and testable model which expose all these connections in a simple framework, in this way taking an advantage of the large amount of available data and still maintaining a parsimonious structure. This task can be accomplished finding meaningful groups of financial and economic time series with similar behaviour in order to model the link between VIX and different informative sources such as oil prices, unemployment and inflation rates and so on.
In particular, the model-based clustering, whose aim is to group a set of objects in such a way that objects in the same group (known as cluster) are more similar to each other than those in other groups, is going to be performed. Standard methods for panel data analysis assume homogeneity across the time series data generating mechanisms. In the above-described specific case, it is of substantial interest to learn if the data generating mechanism is different across the individual time series.
The statistical technique which is going to be used in the present work is using mixture models with time-varying weights, which is much easier to extend to time series data than the methods based on the distance measures.
The majority of clustering analysis in the past researches made use of the methods appropriate for the static data, i.e. the data where the features don't change in time.
In applied statistics, as well as in econometrics a lot of applications deal with relating a random variable Y, which has been observed on several occasions, to a set of explanatory variables through a regression-type model. In my research application I consider as the quantity of interest the volatility index VIX.
However, very often the assumption that the regression coefficient is fixed over all possible realizations is inadequate so the models in which the regression coefficient changes are of great importance. Especially in the cases when these models have to be applied to time series, like in this case to financial and economic data.
The most general alternative is to assume a different regression coefficient for each realization, but only rarely it will be possible to estimate these coefficients without imposing further structure, and modeling them becomes an important point to be considered.
In order to capture the changes in the parameter of a regression model I am using a finite mixture of regression models, also known as switching regression models. They have been mainly studied from a likelihood point of view, i.e. using the procedure for fitting these models by means of maximum likelihood.
In my research I am going to use the Bayesian approach, which aim in contrast to the frequentist approach is not to find the single "best value", but to determine a distribution of plausible estimated values (posterior distribution) for the model parameters.
In fact, in the past decades the mixture regression likelihood function has been often maximized numerically, and several convergence failures are reported for repeated experiments with artificially generated data. Later on, it has been realized that the issue of dealing with an unbounded likelihood function is of significant relevance for heterogeneous mixtures of regression models. In particular, if the variances of a finite mixture of regression models are unconstrained, a global maximizer of the likelihood simply doesn't exist.
So at this point the adopted in my research Bayesian approach reveals to be helpful in this respect.
Thus one of the most important innovative points of my dissertation consists of the fact that in comparison to the major part of the existing mixture models, a dynamic model in order to fit the time-changing data is going to be built.