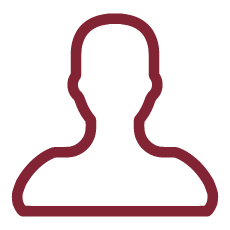
Mixture models have been of intensive interest for the researchers over the last decade. The statistical models which are based on finite mixture distributions capture a lot of specific properties of the real data such as multicollinearity, skewness,kurtosis and unobserved heterogeneity. Mixture models frequently are also referred as semi-parametric models as their flexibility allow to approximate non-parametric problems.
Computationally intensive methods such as Markov Chain Monte Carlo methods and Expectation-Maximization algorithm must be considered. The Bayesian approach using MCMC methods in order to estimate the parameters of interest allows us to transform the complex structure of the mixture model into a set of simple structures using latent variables.
After exploring the existing computational tools for Bayesian inference for finite mixture models, I plan to keep the center of gravity of my research around model-based clustering using mixture models for financial time series. In particular the research question will aim to evaluate the existence of the link between the stock prices' volatility and different sources of information like for example the unemployment rate etc. My purpose thus is to develop a realistic and testable model suitable to investigate such connection, taking in account "non informative" or "conventional" prior distributions depending on the statistical structure of the trial.
In order to adopt a Bayesian approach to model-based clustering using finite mixtures, it is necessary to choose or build a prior distribution that incorporates our subjective beliefs about the parameters. Such priors can be uninformative (completely or essentially) or informative, which represent the actual state of subject-matter knowledge. In my research I aim to keep the center of attention on the "conventional" or "uninformative" priors which don't depend on the available information but on the statistical structure of the trial.
Mixture models in time series offer the opportunity of approximating non-linearities with the advantage that the mixture of simple, possibly linear components, can be much more tractable than non-linear processes. Also mixing is crucial in order to improve forecasting and to detect changes in the time series structure over time.
In my research I plan to implement this type of statistical modeling for financial time series in order to estimate the connection between the volatility of the stock prices and a variety of different information sources. Every such source will have a specific weight,path and distribution, and thus will have a different impact on the final results.