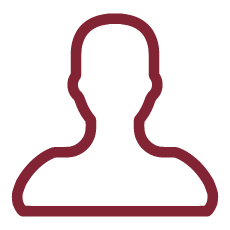
The project deals with the ambitious objective of developing a Machine Learnt model able to predict the erosion/corrosion mechanism in compressor blades subjected to online water-washing techniques for dirt and salt deposit removal. The project will develop along two main research lines: a) the assessment of an innovative mathematical model aiming at predicting the combined effect of erosion/corrosion (pitting) on metal surfaces where deposit fouling can be present due to operating conditions (e.g. due to salt scaling); the model will be implemented on a commercial code (e.g. Ansys Fluent) through an UDF that will be made available on request to any researcher. b) development and validation of a machine learnt approach based on variational auto-encoders and generative adversarian networks able to correctly predict pitting when feeded with the near-wall flow field and droplet characteristics and the blade material.
It is important to point out that these two activities are relevant `per se¿ and will allow obtaining important research outcomes even when they are considered separately.
The development of the project will require a continuous interaction between the two research lines. These will eventually lead to the implementation of one comprehensive tool of the built model that will be able to generalize. In other words, the model will be applied to cases not included in the database, and even in these cases the algorithm is expected to provide accurate predictions. Once developed and validated, this code will represent a powerful tool for supporting companies producing turbomachinery in the definition of the operating conditions (i.e. setting a proper water washing procedure able to guarantee deposit removal) as well as the time lag between successive stops for blading inspection and overhauling.
The recent legislative measures for the sustainable development of energy conversion systems have systematically led to the definition of a new scenario of industrial competitiveness, based not only on energy production (i.e. combined demand for electrical and thermal energy; mechanical drive; waste-to-power), but also on the creation of a virtuous circle, where the performance of the systems is preventively improved starting from the control of the deterioration mechanisms. From an industrial point of view, these strategies generate direct beneficial actions on economic viability, finding fertile ground in the incentives available by environmental protection and innovation programs. The recent legislative measures for the sustainable development of energy conversion systems have systematically led to the definition of a new scenario of industrial competitiveness, based not only on energy production (i.e. combined demand for electrical and thermal energy; mechanical drive; waste-to-power), but also on the creation of a virtuous circle, where the performance of the systems is preventively improved starting from the control of the deterioration mechanisms.
From an industrial point of view, these strategies generate direct beneficial actions on economic viability, finding fertile ground in the incentives available by environmental protection and innovation programs.To the best of our knowledge applications of ML methods to study erosion / corrosion in turbomachinery are quite limited, with most of the real applications being published in the last 3 years.
As for the combined effect of erosion and corrosion (pitting), no paper reports on the development and validation of a reliable mathematical model. For such reason, there is a general consensus about the assumption that the existing models generally lead to an underestimation of the wear over water eroded surfaces, especially when corrosion promotor can be present, e.g. when water washing is performed to remove salt scaling over gas turbine (or offshore wind turbine). Pitting corrosion occurs with extremely localized attacks, called pits or pitting, which penetrate from the surface through the thickness of the metal quickly. The pits, whose dimensions vary from a few tens of micrometres up to a few millimeters (0.1¿m - 2mm), are triggered and propagated in singular points, while most of the metal surface exposed to the environment remains unchanged. The morphologies of the craters are variable: pinpoint, cave, hemispherical. The presence of such craters will enhance the alteration of the surfaces thus increasing losses and reduce structural properties of the blade. The development of an accurate model would allow a proper estimation of the deterioration process, supporting the maintenance of the turbomachinery by properly predicting the operational time between two successive stops for inspection and overhauling.
The use of VANs and GANs seems completely new, and from previous studies this strategy seems to be particularly interesting, as it is able to partially resolve problems related to handling of data for the regression - in fact the encoder can actually handle the surface of the blade being eroded/corroded into a map directly exploiting the computational mesh of the CFD solver. Moreover, through encoding, the computational burden can be largely reduced and finally, given the fact that data are encoded as a normal distribution rather than a single value, VANs can actually be later exploited in GANs training. In a GAN algorithm two neural networks, a generator and a discriminator, are trained against each other in a Nash-equilibrium game. In this, the generator is trained to correctly predict the erosion / corrosion from the flow field and particle description data and the discriminator to correctly classify these generated data as correct or fake. When the algorithm is fully trained, the generator is able to correctly predict erosion / corrosion (and the discriminator to distinguish between correct and fake data). GANs are particularly suited to work with VANs data and their combined use is to the best of our knowledge completely innovative in this field.
We believe that the risks associated with the development of the integrated ML tool can be properly managed due to the existing competences in the research group (as shown below). In any case, we point out that basic models of some of the components to be included in the tool are already existing (e.g. the water droplet erosion model - not including corrosion) and then we can however obtain a somewhat simpler (but comprehensive) version of the tool even if unpredictable difficulties could emerge during the project.