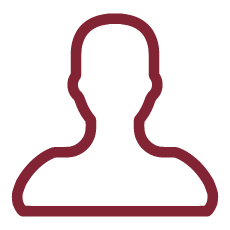
Precision agriculture is a relatively new application field characterized by the use of technology to increase productivity and quality of cultures, while making use of specific policies to preserve the environment. One promising application is mapping.
Precision maps are very essential tool in precision agriculture. They assist growers by showing them the exact locations in the farm and give specific information regarding that location. One major characteristic of a precision map is that it consists of geo-referenced data which is used to show information regarding a precise location in a piece of farm as well as information or characteristic of a soil or a crop like the moisture levels, crop yield soil nutrients levels, crop weed distribution and many more.
There are different types of precision maps that farmer can generate. It enables them to see things they could not spot using their own naked eyes, giving them the ability to make quick decisions which are accurate.
The goal in my research is address this problem of crop / weed mapping, identify and map the crop and weed population in the field from different views (using ground and aerial vehicle) and along different time periods.
The mapping of weed populations allows the monitoring of their evolution, which may contribute to the development of management strategies that reduce the competition period with the culture In this context, the objective was to identify and map the population of weeds in order to use such information in localized application of herbicides.
The robots can also cooperate to generate 3D maps of the environment, e.g., annotated with parameters, such
as crop density and weed pressure, suitable for supporting the farmer's decision making.
Our research build based on two main tasks, First one analysis the field, the second one is generate a map of the fields contains the information extracted from the first step.
In the field analysis we will focus on crop/ weed classification.
Most of research approach build based in machine learning and more specifically Convolutional Neural Networks(CNN), The major drawback of CNNs and FCNs architectures is that the level of expressiveness is limited by the size of the training dataset. In the context of precision farming, collecting large annotated datasets involves a significant effort. Datasets should be acquired across different growth stages and weather conditions.
Although the methods described so far can successfully mitigate the annotation effort issue, they may not yet achieve the segmentation performance of a fully trained FCN. Also all the above approaches build based on one view(ground or aerial ). In this direction we propose two main approaches, first we aim to build two architectures build based on deep learning with multiple input, the first architecture for ground vehicle, the second one for aerial vehicle, and then we aim to use the two view to make better analysis for field, that allow us to generalize well to different crop and weed, also we aim to generate synthetic dataset using machine learning approach. More recent approaches make use of GANs. Giuffrida et al.[1] exploit a conditional GAN to generate 128×128 synthetic Arabidopsis plants, with the possibility to decide the desired number of leaves of the final plant. Their method has been tested by using a leaf counting algorithm showing how the addition of synthetic data helps to avoid over-fitting and to improve the accuracy. In [2], the authors leverage a GAN to generate artificial image samples of plant seed lings to mitigate for the lack of training data. The proposed method is capable to generate 9 distinct plant species while increasing the overall recognition accuracy. Unlike the last method, in this work we propose to generate multi-spectral views of agricultural scenes by synthesizing only the objects that are relevant for semantic segmentation purposes.
The second aspect in our research is build a map from two views aerial-ground,. In this direction there is not too much approaches in the state of the art mapping works. We propose to build a collaborative 3D Mapping pipeline, which provides an effective and robust solution to the cooperative mapping problem with heterogeneous robots, specifically designed for farming scenarios.
REFERENCES:
[1]Valerio Giuffrida, M., Scharr, H., Tsaftaris, S.A.: Arigan: Synthetic arabidopsis plants using generative adversarial network. In: Proceedings of the IEEE International Conference on Computer Vision Workshops. pp. 2064-2071 (2017)
[2]Madsen, S.L., Dyrmann, M., Jørgensen, R.N., Karstoft, H.: Generating artificial images of plant seedlings using generative adversarial networks. Bio systems Engineering 187, 147-159 (2019),http://www.sciencedirect.com/science/article/pii/S1537511019308190