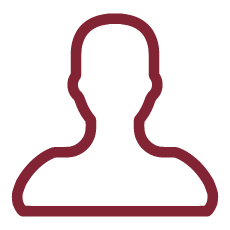
The aim of this research project is to develop a Mixed Hidden Markov Model for joint estimation of multiple quantiles in a multivariate linear regression for longitudinal data. This method accounts for association among multiple responses and study how the relationship between dependent and explanatory variables may vary across different quantile levels of the conditional distribution of the multivariate response variable. Unobserved heterogeneity sources and serial dependence are jointly modeled through the introduction of individual-specific, time-constant random coefficients and time-varying parameters that evolve over time with a Markovian structure, respectively. Estimation of model parameters is carried out via a suitable EM algorithm without parametric assumptions on the random effects distribution.
Longitudinal data allows us to understand the evolution of a certain phenomenon across time. In this context, it becomes of crucial importance to determine an appropriate modelling framework to assess the effects of unobserved factors and hidden heterogeneity which can be either time-invariant or time-varying; ignoring these factors may induce a strong bias and lead to false conclusions of the study. To account for longitudinal data features, including serial heterogeneity, the MHMM accommodates both such sources of random variation by considering unobserved heterogeneity in the model parameters via individual-specific random coefficients; both covariates and random effects are involved to capture differences among processes. This research proposal tries to combine the salient features of quantile regression and MHMMs for multivariate longitudinal data analysis. The former allows for quantile-specific determinants of different responses and by jointly modelling them, we can borrow information across responses and conduct joint inference at the marginal quantiles level, revealing some possible underlying truth that can not be detected by univariate models. The latter has the advantage that different sources of heterogeneity can be distinguished: between individual heterogeneity and time heterogeneity modelled through the state-specific effects.