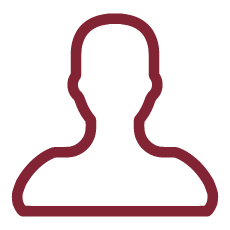
The research project aims at the use of computational intelligence techniques, neural networks and fuzzy logic, in a real context of prediction of energy series, to implement a system for optimal management of renewable energy sources in smart grids.
Distributed scenarios are increasingly common in which small production units are connected directly to the distribution network. Economic, environmental and incentive policies have pushed the energy market towards distributed renewable sources. In these contexts, however, the greatest difficulties are due to the variability and reliability of resources. For an efficient management of the whole system, the prediction of the energy produced becomes necessary so that an intelligent adaptation of the distribution network is possible. This is made possible by the fact that the production parameters of any generation unit are constantly monitored with smart metering technologies that give the possibility to obtain current and output voltage in real time.
In the prediction of time series the non-stationarity and, often, the non-linearity of the series themselves lead to complex dynamics, with chaotic properties, which are difficult to model adequately using standard predictive models. This project therefore aims to adapt machine learning techniques to analyze data associated with renewable energy sources and intellingently adapt it to the management of smart, possibly isolated, smart grids. In particular, the project aims to develop solutions that can make use of machine learning algorithms for prediction in totally distributed systems in which heterogeneous agents interact (large photovoltaic plants, domestic cogeneration, etc.) with the constraint of controlling all the RESs and their surplus storage.
Innovatività della ricerca, e potenzialità di realizzare un avanzamento delle conoscenze rispetto allo stato dell'arte
In this research, we focus the attention on short-time prediction, with a time horizon of three days. This choice is made because we found it to be the most useful in the real application case, where the management system can optimize the BESS state of charge based on the forecasting of the load and the prediction of the solar production, minimizing losses. The developed techniques could also be used with different time horizons for different applications in diverse frameworks. In literature we can find statistical models [1-3], stocastic predictors [4,5], fuzzy systems [6], neural networks [7], artificial intelligence-based neural networks [8].
Some articles present also the comparison between predictions obtained with methods based on two or more prediction techniques [9]. The literature is wide on this subject but only few works describe in detail the prediction models that can be used for daily prediction in PV plants. Based on past measurements and weather forecasting, power is estimated via ANN [10] or soft computing techniques [11], while in [12] ANN is applied only to past samples. ANNs can also be used in addition to numerical models for weather prediction (NWP) based on satellite imaging [13]. This models are also used for Multi-Model Ensemble techniques (MME) with output power prediction [14]. Other, more complex, forecasting schemes are proposed in [15] where ANN is used to improve standard prediction models such as ARMA.
The innovative idea of this research is adapting new learning paradigms for renewable energy sources management, starting from techniques used in literature applied to other fields such as risk management, biomedical engineering and econometrics, and applying them to PV prediction and BESS management. The stochastic and highly chaotic behavior of the solar energy time series requires in fact to implement ad-hoc procedures for this type of data, which will be developed by working only on the past measurements of the electrical quantities.
[1] Hammer, A.; Heinemann, D.; Lorenz, E.; Lückehe, B. Short-term forecasting of solar radiation: a statistical approach using satellite data. Solar Energy 1999, 67, 139 -150.
[2] Safi, S.; Zeroual, A.; Hassani, M. Prediction of global daily solar radiation using higher order statistics. Renewable Energy 2002, 27, 647-666.
[3] Lorenz, E.; Hurka, J.; Heinemann, D.; Beyer, H.G. Irradiance forecasting for the power prediction of grid-connected photovoltaic systems. IEEE J. Sel. Top. Appl. Earth Obs. Remote Sens. 2009, 2, 210.
[4] Kaplanis, S.; Kaplani, E. A model to predict expected mean and stochastic hourly global solar radiation values. Renewable Energy 2007, 32, 1414 - 1425.
[5] Kaplanis, S.; Kaplani, E. Stochastic prediction of hourly global solar radiation for Patra, Greece. Applied Energy 2010, 87, 3748 - 3758.
[6] Iqdour, R.; Zeroual, A. Prediction of daily global solar radiation using fuzzy systems. Int. J. Sustainable Energy 2007, 26, 19-29.
[7]. Kemmoku, Y.; Orita, S.; Nakagawa, S.; S.akakibara, T. Daily insolation forecasting using a multi-stage neural network. Solar Energy 1999, 66, 193 - 199.
[8] Hocaoglu, F.O.; Gerek, O.N.; Kurban, M. Hourly solar radiation forecasting using optimal coefficient 2-D linear filters and feed-forward neural networks. Sol. Energy 2008, 82, 714-726.
[9] Zervas, P.; Sarimveis, H.; Palyvos, J.; Markatos, N. Prediction of daily global solar irradiance on horizontal surfaces based on neural-network techniques. Renewable Energy 2008, 33, 1796 - 1803.
[10] Mellit, A.; Pavan, A.M. A 24-h forecast of solar irradiance using artificial neural network: Application for performance prediction of a grid-connected {PV} plant at Trieste, Italy. Solar Energy 2010, 84, 807 - 821.
[11] Voyant, C.; Muselli, M.; Paoli, C.; Nivet, M.L. Optimization of an artificial neural network dedicated to the multivariate forecasting of daily global radiation. Energy 2011, 36, 348 - 359.
[12] Sfetsos, A.; Coonick, A. Univariate and multivariate forecasting of hourly solar radiation with artificial intelligence techniques. Solar Energy 2000, 68, 169 - 178.
[13] Mellit, A.; Kalogirou, S.A. Artificial intelligence techniques for photovoltaic applications: A review. Prog. Energy Combust. Sci. 2008, 34, 574 - 632.
[14] Barbieri, F.; Rajakaruna, S.; Ghosh, A. Very short-term photovoltaic power forecasting with cloud modeling: A review. Renewable Sustainable Energy Rev. 2017, 75, 242 - 263.
[15] Pierro, M.; Bucci, F.; Felice, M.D.; Maggioni, E.; Moser, D.; Perotto, A.; Spada, F.; Cornaro, C. Multi-Model Ensemble for day ahead prediction of photovoltaic power generation. Solar Energy 2016, 134, 132 - 146.