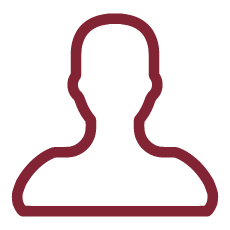
The project deals with regression models for multivariate mixed responses. When a proper multivariate model is not available, as it is often the case with mixed responses, the approach is usually that of defining a (conditional) regression model for the univariate profiles and linking such profiles through a structure of dependence. This can be either based on joining profile-specific random effects through a parametric or semiparametric multivariate distribution, as in Gueorguieva (2001), Chib & Winkelmann (2001) or through copula models, describing the association between different profiles, as in Zimmer & Trivedi (2006). We consider the first option and, within this framework, we develop a class of flexible models by means of discrete, outcome-specific, latent effects, used to account for potential dependence between outcomes. The structure of dependence, whose complexity grows exponentially with the numbers of profiles, can be approximated by tensorial methods designed for multiway data, such as the Tucker or Candecomp/Parafac models. In particular, the latter model appear to be very promising due to its interesting properties. Namely, the Candecomp/Parafac model synthesizes a tensor through a limited number of latent factors, providing the best tensorial rank approximation of the observed tensor, and the solution is unique under mild conditions. In the domain of regression models for multivariate mixed responses, we are interested in using possibly different numbers of locations in each response, joined by a full association structure. The Tucker3 or Candecomp/Parafac models might be used in order to summarize such a full association structure, providing a parsimonious model in terms of the number of parameters.
References:
See Section "Inquadramento della ricerca proposta in ambito nazionale ed internazionale".
The research project has several goals involving the development of new statistical regression models for multivariate mixed responses. The most relevant source of innovation relies in the attempt to relate two quite different fields of research. As far as we know, no interactions between the huge literature on regression models for multivariate mixed responses and that on tensor models exist. In the project we will try to use tensor methods for enhancing the interpretation of latent effect regression models for multivariate mixed responses.
The results we will discover will be presented at international and national conferences and will be submitted to international statistical journals with impact factor and belonging to the A-class.
Particular attention will be paid to the practical implementations of the new models. The open-source statistical software R will be adopted and new R functions and packages will be released. For this purpose, the R package ThreeWay (Giordani et al., 2014) can be used for finding the tensor model solutions.
Reference
Giordani, P., Kiers, H.A.L., & Del Ferraro, M.A.: Three-way component analysis using the R package ThreeWay. Journal of Statistical Software 57, 1-23 (2014).