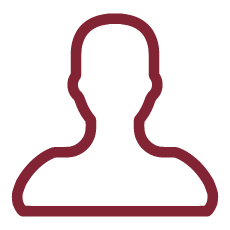
This study analyzes different parametric and non-parametric modeling methods for estimating the Loss Given Default (LGD) of bank loans for shipping finance. The shipping industry is considered the backbone of global trade and the global economy but it is associated with several risks which create the need for a more detailed loss modeling from the bank perspective. LGD is the amount of money a financial institution loses when a borrower defaults on a loan, expressed as a percentage of total exposure. For this study, we will use a unique database of defaulted loans in European banks that are involved in shipping finance. The main goal of this study is twofold: to compare the performance of alternative LGD modeling methodologies in shipping finance and to provide some insights into what drives LGD in the shipping industry. To achieve this, the research study will be divided into two main parts. First, we will compare the performances of traditional statistical parametric models with a wide set of machine learning algorithms including bagged trees, random forest, boosted trees, support vector machines, and multivariate adaptive regression splines (MARS). Secondly, we will apply a variable importance measure built on the idea of the permutation importance, to analyze the risk drivers with the greatest effects on the LGD for shipping finance prediction accuracy for each method. In this regard, we further explore what features drive the results of each algorithm's prediction. Therefore, in this way, we first identify the best forecasting method in the shipping-related transactions, as well well go beyond this and throw some light on the popular perception of the "black box" nature of machine learning decisions.
LGD in shipping finance still remains an unexplored topic in the academic literature and the lack of data availability remains the main reason. There are few studies about bank loans of shipping finance but they are mainly focused on analyzing the factors to assess and predict the default risk involved in shipping loan agreements. As all of the existing studies mainly focus on default risk in shipping loans, there are no empirical studies concerning the LGD of bank loans in shipping finance. To the best of my knowledge, this study is the first one that comprehensively investigates estimation and prediction methods for shipping finance LGD as well as trying to provide some new insights into what drives LGD in the shipping industry.
The main innovation of our study stands in comparing different parametric and non-parametric estimation techniques, including here recent innovation machine learning algorithms, of LGD in the shipping industry. This will offer banking practitioners new tools to consider when they are building internal models for borrowers in the shipping industry. This research will provide interesting findings into the level and distribution of LGD in shipping finance as well as identify the main influencing factors. A special focus in this study will be given to the underlying collateral structure in order to reveal information on the relationship between different vessel types and LGD across models. The findings will be useful for bank practitioners when building internal advanced models for the estimation of loan losses.
Finally, the use of recent popular tools that are popular in the field of explainable machine learning such as permutation ranking importance or partial dependence plot that we will use in our study, reveal important implications and insights for the practitioners that want to include these innovative algorithms in their future models.