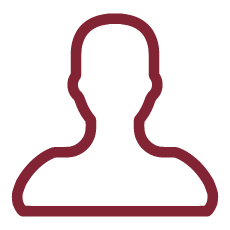
The project aims to develop and implement a general methodology for the aggregate calibration of transport network models, which exploits different data sources and is suitable to deal with individual data collected in mobility, like Floating Car Data, jointly with other data sources within a multi-step optimization procedure based on metaheuristic algorithms. The application of the methodology will be tested in a case study concerning the update of the Traffic Model of Rome with new data collected in mobility.
The application fields of the proposed methodology for aggregate transport network models calibration will be broad and will cover different types of models, independently of their specific formulation and extent. The crowdsourcing of individual data collected in mobility is today a suitable source of data for updating transport system models and will be even more widely available in the future. These technological advances open even broader perspectives for the application of aggregate calibration based on crowdsourcing of mobility data.
The aggregate calibration of a transport network models is usually formulated as an optimization problem consisting of minimizing an error function with respect to the coefficients of the model, given a set of traffic counts and the corresponding simulated values, also given the values of the variables describing the activity system and the transport network supply model. The availability of many sources of information from ITS applications enables using even different variables from traffic counts, like speed, density, and travel times, which characterize the transport network performances and exploit observed users¿ trips.
Among the metaheuristic algorithms, the Particle Swarm Optimization (PSO) algorithm will be proposed because, unlike genetic algorithms, it is defined on continuous variables and allows exploring a wide range of the feasibility region.
PSO algorithm is a stochastic optimization technique inspired by social natural organisms¿ behavior, like ants and bees, and applies a collective strategy to reach the minimum value of the objective function. Each individual of the swarm moves according to a strategy that combines different rules: random steps and movements toward social and individual previous bests. The system is initialized with a population of random solutions and searches for optima by updating generations. In PSO, the potential solutions, assimilated to particles of a swarm, move through the feasible region of the problem space. Each particle¿s movement direction and speed depend on a combination of the distance of its current position from its individual best and the current collective best of the swarm. The iterative searching procedure continues constrained within the boundaries of the feasibility set until maximum iterations, or minimum error criteria are not attained. The choice of the feasibility region is an essential issue of the procedure. It enables the analyst to impose constraints on the variables that reflect the statistical properties of the variables, the physical nature of the problem, and the engineering aspects to take into account.
The crowdsourcing of individual data collected in mobility is today a suitable source of data for updating transport system models and will be even more widely available in the future. These technological advances open even broader perspectives for the application of aggregate calibration based on crowdsourcing of mobility data.
As it has been highlighted in the state of the art section, no advanced methodology has been developed until now for the aggregated calibration of macroscopic traffic models using individual mobility data which were instead used for microscopic models.
Since macroscopic models are anyway the most sound methodology for online applications and traffic forecasting the expected impact of the research is highly significant.