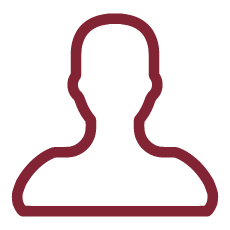
The herein proposed DESIGN (Distributed Evolutionary Swarm Intelligence and Granular Computing Techniques for Nested Complex Systems Modelling) project aims in defining, developing and implementing a general framework for complex systems modelling. Our basic hypothesis is that searching for regularities in data coming from the input-output sampling of the process to be modeled can be carried out by a set of agents' swarms, in charge to perform a hierarchical information granulation. Each agent performs a clustering procedure, or even more advanced information granulation tasks, on subsets of entities belonging to the previous level. An evolutionary computation orchestration coordinates the swarms in finding pivotal information granules (symbols) extracted automatically from the training set, aiming to identify suitable embedding spaces where the final classification models can be trained. The whole synthesis procedure is driven by a performance measure computed on a validation set. A backtracking mechanism, supported by consensus procedures and based on a penalty/reward strategy, is in charge to update the fitness of each information granule, as well of agents that contributed to spawning the fittest granules.
The whole machine learning algorithm is conceived to deal directly with unconventional, structured domains, such as fully labeled graphs and sequences, considered herein as the most suitable way to gather samplings coming from complex systems.
DESING aims in developing a software library for rapid application development of complex systems modelling algorithms. In order to test the effectiveness of the proposed machine learning approach, three different vertical applications will be tackled, coming from the areas of cybersecurity, bioinformatics and precision medicine, predictive maintenance on power grids.
To the best of our knowledge, no machine learning approach granting all the desired features listed in the previous section is currently available to the machine learning community.
Beside the innovation brought by the novel proposed approach to complex systems modelling, DESIGN will focus on the development of a software API library, as well as vertical executables, each one dedicated to the following research topics.
a) Real-time identification of security attacks in Wi-Fi networks
Modern information systems deeply rely on cyber and communications security techniques in order to protect the information itself and guarantee its correct delivery to remote endpoints. The high data rates achieved by modern hardware in terms of both processing and transmission of information, make the security analysis not only a complex task but also a big data problem [1]. Furthermore, the complexity of communication networks and the structured patterns they generate, require machine learning techniques able to act on sequences of objects in order to exploit features not observable on single data packets [2]. Protocols themselves are even more oriented to work in streaming contexts, in order to let network devices to apply traffic engineering procedures more efficiently.
Evolutionary algorithms can drive changes in algorithms' setup parameters such that knowledge extraction capabilities of such algorithms can be maximized by looking for recurrent subsequences at different levels of information granulation. This kind of approach makes possible to conceive new kind of algorithms able to understand how communication protocols work and how to exploit their key features in order to evaluate how effective security (counter-)measures are.
b) Mining biological networks for precision medicine
The vast majority of the information in biological systems is encoded in the way atomic units interact one another. This is why graphs are widely used to model complex biological systems and the need for designing advanced structural machine learning techniques able to capture semantically relevant motifs is evident. Metabolic networks, for example, model the chain-like nature of chemical reactions within cells and finding clusters of (functionally) similar micro-organisms, especially in the gut flora, would allow the profiling of "healthy" subjects [3]. On the other hand, sick patients can be characterized by matching their profiles with healthy subjects, fostering the development of low-cost target drugs (balanced drugs containing properly chosen micro-organisms mixture) in order to restore the optimal gut flora equilibrium, crucial to avoid systemic diseases [4].
Protein residue networks are another example of complex biological system described as a 3D graph sketching the folded state of a protein. By mining for relevant amino-acid complexes or motifs, one can unravel the allosteric zone(s): the main actors for the allosteric effect (which can only be found in enzymatic proteins). Indeed, precision drugs working on the top of the allosteric zone, due to the enhanced signaling pathway, are much more efficient than standard drugs operating on the active site of a protein [5].
c) Fault Recognition and Predictive Maintenance Systems on Power Grids
To overcome the limitation of already existing research application [6] (in collaboration with AReti S.p.A.) related to Condition Based Maintenance applications and, specifically, to a fault detection system for medium voltage lines able to process power grids measurements in terms of different characteristics, including categorical, metric and time series of short breaks, it is of paramount importance the development of a general framework in charge of finding local regularities for describing specific sub-classes of faults. In this application, DESIGN, is the fundamental framework for modeling the fault phenomenon characterized by a local and time-varying nature, aiming to minimize the total cost of inspection and repair, by gathering and interpreting heterogeneous data related to the operating condition of the network and its components.
[1] Bhuyan, M. H., et al. (2013). Network anomaly detection: methods, systems and tools. IEEE Commun. Surv. Tutorials, 16(1), 303-336
[2] Kolias, C., et al. (2017). TermID: A distributed swarm intelligence-based approach for wireless intrusion detection. Int. J. Inf. Secur., 16(4), 401-416
[3] Cani, P. D. (2018). Human gut microbiome: hopes, threats and promises. Gut, 67(9), 1716-1725
[4] Marchesi, J. R. et al. (2016). The gut microbiota and host health: a new clinical frontier. Gut, 65(2), 330-339
[5] Csermely, P. et al. (2013). Structure and dynamics of molecular networks: a novel paradigm of drug discovery: a comprehensive review. Pharmacol. Ther., 138(3), 333-408
[6] De Santis, E. et al. (2015). Modeling and recognition of smart grid faults by a combined approach of dissimilarity learning and one-class classification. Neurocomputing, 170, 368-383