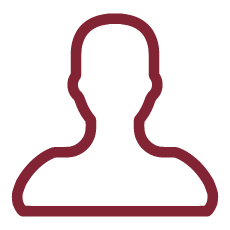
Nowadays, in almost any research field data are inherently complex and high dimensional, due to the increasing availability of information granted by the new technologies. Classical approaches to supervised and unsupervised statistical learning are inadequate and cannot be directly applied to these "modern" data. The inadequateness is due to the increased complexity of:
a) data schemes. The classical data scheme "statistical units x quantitative variables" is often obsolete. Newer and more informative schemes are in use: mixed data, where some variables are quantitative and some other are categorical, matrix data, where the same units (persons or objects) are measured on the same variables in different occasions, multidimensional networks, where multiple relations are observed among a set of units (nodes) etc.;
b) models. New technologies make available a huge amount of features (variables) for the same unit. Classical methods do not allow us to model properly such high dimensional data because they would produce models with a large number of parameters that cannot be efficiently estimated, especially when the number of observations is small compared to the number of parameters and/or variables;
c) algorithms. On high dimensional data, the estimation and/or selection of classical models become computationally infeasible and/or do not give the results in the required time.
The aim of the research group is to work on the aforementioned three sources of complexity proposing new statistical learning methods for unsupervised classification able to:
1) face with new complex data schemes: mixed data, matrix data and multidimensional networks;
2) model parsimoniously. The idea is to introduce new flexible parameterizations that are sufficiently parsimonious and allow us to select the relevant information when the number of variables is very large.
3) reduce the computational complexity of the algorithms making use of new methods of estimation and/or model selection.
The aim of the research is to innovate and progress beyond the state of the art along several directions in the field of unsupervised classification. The first direction is to propose new parsimonious reparameterizations for the finite mixture of Gaussians (FMG) model. An idea is to assume that the covariance matrix of each component has an ultrametric structure (Cavicchia et al., 2020). This corresponds to parameterize the covariance matrix by a small number of parameters equal to the number of variables and it can be interpreted as a hierarchical classification of the variables. In this way, we obtain several partitions of the variables, one for each component, i.e. for each cluster of the units' partition. Another idea is to assume that the class conditional covariance matrices follow a Canonical Decomposition (Carroll and Chang, 1970). This corresponds to assume that each matrix is a linear combination of the same set of rank 1 symmetric matrices. Model selection reduces to the selection of the number of rank 1 matrices. The last reparameterization of the FMG model regards its extension to the three-way case. It is interesting to note that in this setting the set of mean vectors can be seen as a three-way array of the form components x variables x occasions. Our idea is to reduce the complexity of the model by applying on such an array the well know three-way component models like Tucker (Tucker, 1966; Vichi et al., 2007) or Parafac (Harshman, 1970; Giordani and Rocci, 2017). Such models were proposed and developed by several authors (Kroonemberg, 2008), as possible extensions of principal component analysis to three-way arrays.
The second direction of research is the extension of the model proposed by Ranalli and Rocci (Computational Statistics and Data Analysis, 2017) to cluster mixed type data to the case of three-way data where the different occasions are given by points in time. In this way, a hidden Markov model is obtained. A further extension of this model will be to the case of biclustering (Martella and Alfò, 2017), where the interest is to provide a joint clustering of units and variables. The task is also known under a broad range of different names, including double clustering or co-clustering. The idea is to partition the data matrix into homogeneous blocks with respect to some observed features. Each block is given by a subset of units, e.g. individuals, and a subset of variables, e.g. responses to a questionnaire. Two individuals, belonging to the same block, give similar answers not only to the same question but also to different questions of the same block.
The third direction is to explore the possibility to simplify the search of the best model when fitting a finite mixture of linear regression models. In particular, our attention will focus on the calibration of the tuning parameters that regulates the weight of the LASSO penalty by exploiting the properties of the LARS algorithm (Efron et al., 2004).
The fourth direction is about multidimensional network data, which can be considered as three-way, two-mode, data. Our goal is to build a clustering framework for multidimensional networks based on infinite mixtures in a latent space framework. We assume that the latent coordinates of the nodes are distributed according to an infinite mixture of Gaussians. This prevents a major issue in clustering: the choice of the number of clusters. Edge probabilities, in the case of binary multiplexes, can be defined via a logistic function of the nodes coordinates in the latent space as well as other network specific parameters (D'Angelo et al., 2020). The latent space addresses both the clustering behaviour of the networks and the transitivity, as in simpler latent space models for network data. Instead, the network-specific parameters provide a flexible and parsimonious model for the edge probabilities.
The fifth direction of innovation will be the application of our proposals to real data.
Carroll J.D., Chang J.J. (1970). Analysis of individual differences in multidimensional scaling via an N-way generalization of "Eckart-Young" decomposition. Psychometrika, 35, 283-319
D'Angelo S., Alfò M., Murphy T.B. (2020). Modeling node heterogeneity in latent space models for multidimensional networks, Statistica Neerlandica, to appear, https://doi.org/10.1111/stan.12209
Efron B., Hastie T., Johnstone I., Tibshirani R. (2004). Least angle regression. The Annals of Statistics, 32(2), 407-499
Harshman R.A. (1970). Foundations of the PARAFAC procedure: Models and conditions for an "explanatory" multi-modal factor analysis. UCLA Working Papers in Phonetics, 16, 1-84
Kroonemberg P.M. (2008). Applied multiway data analysis. Hoboken, NJ: Wiley
Tucker L.R. (1966). Some mathematical notes on three-mode factor analysis. Psychometrika, 31, 279-311
Vichi M., Rocci R., Kiers H.A.L. (2007). Simultaneous Component and Clustering Models for three-way data: within and between approaches. J. of Classific., 24: 71-98