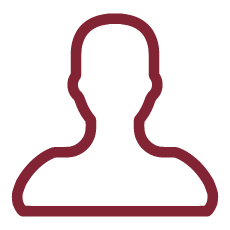
Knowledge of the pressure field in water distribution networks (WDN) is important because it generally drives the operational actions for leakage and failure management, backwater intrusion and demand control. This knowledge would ideally be achieved by monitoring the pressure at each junction of the network. However, due to limited economic resources, only a small number of nodes can be controlled. Therefore, in order to obtain complete information on the pressure field while containing monitoring costs, two different steps will be followed in this research. First, several optimal sampling design methods will be studied, in particular entropy-based methods and methods based on the variance-covariance uncertainty matrix. In both cases, the optimal solution will be sought through single-objective (SOGA) or multi-objective (MOGA) genetic algorithms models. Second, the pressure values of the optimal groups (i.e. the optimal solutions from sampling design) will be used as input of an ANN which will generate as output the pressure values at all the other non-monitored nodes of the system. In sampling design and ANN training a pressure-driven hydraulic model with demand scenarios [ ] derived from the scaling laws of water consumptions [] will be used.
The progressive depauperation of underground water resources, due in great part to the anthropic pressure, and the increasing incidence of pollution phenomena due to the presence of productive activities all over the territory, are some of the elements that make necessary a reformulation of water resources management policies. These issues, whose mitigation, if possible, involves great financial investments, have pointed out the opportunity to rationalize the entire production process, both binding the price of the resource to its effective production cost, and diminishing wastes and losses. In this context the problem of the location and the reduction of losses in water distribution networks assumes particular importance. In Italy physical losses are estimated to be larger than 30% of the volume entering distribution systems and most of them are background losses, that is small losses difficult to discover. They are due both to the state of degradation in which great part of distribution systems unfortunately pours, and to their bad management. They produce great financial losses, and a huge, even if not quantifiable environmental damage. In this context this research is aimed to support the more traditional policy of losses localisation and elimination with the policy of their reduction, especially in those cases, like in water distribution networks, where a direct intervention would be too expensive as well as full of technical difficulties. An inexpensive and efficient method for physical losses reduction is the optimization of pressure heads and their maintenance on the values strictly necessary to guarantee the efficiency of the service. First of all a set of measurement devices should be installed for transmitting in real time, through a telemetering system, the time evolution of heads in relation to the variability of the demand. It is obvious that the number of instruments should be as small as possible in order to limit the metering system cost. Consequently, the values of hydraulic heads all over the network must be reconstructed from that little number of measurements available. In this research a methodology for the reconstruction of the time evolution of pressure heads in a real water distribution network is presented, estimating the reliability of the results under dynamic conditions of water demand. The knowledge of pressure heads in a time step all over the network can be then employed in the formulation of an optimal valves management in order to obtain pressures reduction and therefore the control of physical losses.
Moreover, one of the main and innovative focus of this research will lie on interpreting the water demand as a probabilistic parameter in WDS models and on the use of the scaling laws as tools able to describe demand statistics at different scales. Moreover, the optimization models for the design and management of WDS are usually based on the assumption of perfectly defined working conditions, leading to solutions which are optimal for the considered inputs, but may be unreliable if reality turns out to be different. The inclusion of uncertain consumptions in network design and management optimization problems is then a challenging task.