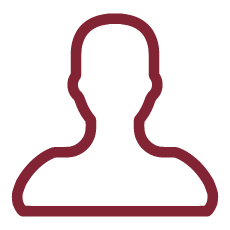
The last financial crisis emphasized the fact that the consequences of credit risk management could affect not only one financial institution but also the entire financial system and going beyond to the global economy.In response to the last crisis, the Basel Committee on Banking Supervision and the Bank of International Settlement proposed a set of banking regulations referred as Basel II to quantify their capital requirements for credit risk. Loss Given Default (LGD) is one of the main credit risk parameters that has to be carefully evaluated by banks. The challenge of finding an effective forecasting methodology for Loss Given Default as a critical component in credit risk management, is increasing in importance. The improvements in prediction accuracy are essential for lenders and investors for a better assessment of credit risk and pricing strategies while estimating possible future credit losses. Loss Given Default presents the credit loss incurred if a counterparty of a bank defaults and is one of the key elements in the framework of Basel II for calculating banks regulatory capital requirements. Recent studies have emphasized the use of innovative algorithms as an alternative interesting forecasting tool in the LGD topic.The use of these algorithms is a new research area in this field and still remains limited since there are several algorithms that have confirmed to be successful in other fields which can be implemented. In this spirit, this study aims to explore different forecasting algorithms with the aim to improve the accuracy when predicting LGD as a critical component in the credit risk framework.
Related to research questions presented above, the main contributions and innovations of this study consist as following:
First, we will focus on identifying risk drivers for LGD modelling with the goal to improve the currently existing models in the literature. In this framework, we intend to perform the use of machine learning techniques and algorithms to analyze variables selections and see whether they are a good alternative for LGD modelling. Our goal is to present improved models that give substantial increases in predictive accuracy compared with the previously published methods of machine learning techniques. To the best of our knowledge there is no other study that compares the predictive performance of methods mentioned above for the LGDs of defaulted loans.
Secondly, there exist only few studies on defaulted bank loans and they are usually based on short samples or focus only on a single country or a loan type. The majority of the existing literature consists of empirical studies for corporate bonds. This is mainly related to the difficulty of finding the available data. We instead will use a unique and rich data set that provides defaults for different countries and also various loan types.
Finally and most important, we aim to emphasize some practical considerations about the implementation of specific Machine Learning techniques for LGD models among banks. In addition this study will present the used models and their outputs in a simpler and understandable way so it can be accepted and effectively put into production by banks. We believe that our research will derive recommendations for practitioners in order to get accurate forecasts for LGD with high predictive power in the future.