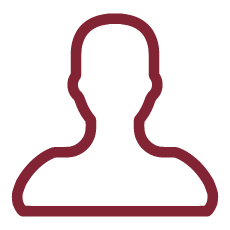
In parametric models, there are several reasons why the estimators can be biased and inconsistent.
We will focus on two sources of bias, namely measurement error in the dependent variable and unrepresentative samples, and we will propose an estimation method that simultaneously corrects for this double source of bias. Most empirical work is based on observational data that are unrepresentative
of the population of interest. Sample selection models attempt to correct for non-randomly selected
data in a two-model hierarchy where, on the first level, a binary selection equation determines if a particular observation will be available for the second level (outcome equation). In the case of binary choice models, we will assume that also the dependent variable of the outcome equation is binary. The likelihood function takes into account the selection mechanism and allows for unbiased
parameters estimation. We will extend this framework to the situation of a measurement error in the
dependent variable of the outcome equation. We will use a parametric approach to the estimation of the
probabilities of misclassification by incorporating them in the likelihood of a binary choice model with sample selection.
To our best knowledge, there is no work in the literature to have tackled simultaneously the problem of misclassification of the dependent variable in binary choice models and that of sample selection. Still this situation occurs quite often in the literature. One relevant field, which will represent the applied part of our research, is the field of undeclared work.
Undeclared work, in the sense of any paid activities, which are legal but concealed from public authorities, is an important component of tax and social security fraud. There is a legitimate concern that today¿s difficult labour market and social situation encourage undeclared work. Furthermore, in the context of European economic recovery, undeclared work has become a true challenge for the labour market policies, mainly because it affects tax revenue, social security and labor standards. Without better understanding of the main determinants of undeclared work, little can be done to prevent it. With this aim in mind we will use the Eurobarometer survey conducted on the 28 EU countries in 2013. We will use the following question as the dependent variable of our model ¿Apart from a regular employment, have you yourself carried out any undeclared paid activities in the last 12 months?¿. The problem of selection bias arises because there is a vast amount of non-response (around 60%) and the missingness mechanism is clearly not completely at random; the problem of misclassification arises because it is very likely that someone who is working off the book, denied his/her involvement.