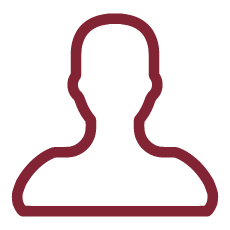
Today, big data, genomics, and quantitative in silico methodologies integration, have the potential to push forward the frontiers of medicine in an unprecedented way. Clinicians, diagnosticians and therapists have long striven to determine single molecular traits that lead to diseases. What they had in mind was the idea that a single golden bullet drug might provide a cure. Unfortunately, individual diseases rarely share the same mutations. This reductionist approach largely ignores the essential complexity of human biology. Indeed, a large body of evidence that is now emerging from new genomic technologies, points out directly to the cause of disease as perturbations within the interactome, i.e. the comprehensive network map of molecular components and their interactions. As a matter of fact, a growing body of knowledge reveals the association between groups of interacting proteins and dis-ease within the so-called human interactome, representing the cellular network of all physical molecular interactions.
The project will focus on three main questions:
- Using molecular networks to understand the relationships between COPD susceptibility genes.
- Identification of molecular networks related to COPD pathogenesis: extend previous works to gene regulatory and protein-protein interaction networks.
- Utilization of molecular networks to understand COPD heterogeneity.
The new general framework for network medicine which integrates methods from graph theory and machine learning is particularly suitable in the contest complex of personalized medicine for complex respiratory disease precision. The large amount and heterogeneity of molecular data and the effective formulation of medical problems based on large-scale data in terms of the topology of the network, bring a significant advancement with respect to results in the literature.
The new general framework for network medicine which integrates methods from graph theory/ combinatorial optimization and machine learning, is considerably more complex and general than those used so far. This complexity allows us to cover, in a mathematically sound way, different problems arising in COPD precision treatment. The coupling of both methodologies (graph and machine learning theory and applications) is particularly suitable in the contest of network medicine for precision oncology given the large amount and heterogeneity of molecular data and the effective formulation of medical problems based on large-scale data in terms of the topology of the network, thus bringing a significant advancement with respect to results in the literature. Moreover, network representations coupled with the pattern identification power of machine learning techniques are the ideal tools for representing relationships among data, finding "patterns" for biomarker studies and, most importantly, any kind of expertise can find the opportunity to fully express itself using a common language. In fact, this working framework provides plenty of words and concepts that can be exploited by biologist/clinicians to represent their knowledge and, by bioinformaticians or computational modelers, to derive algorithms and find biological/medical answers using computation.