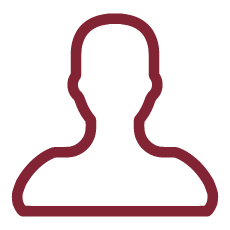
The aim of the research is to design and optimize multimedia underwater sensor networks for smart monitoring of remote marine energy generation plants made of underwater turbines exploiting sea currents for generating energy.
By relying on a combination of research ideas on machine learning-based optimisation of underwater wireless networks (to transmit in real-time monitored data) as well as on processing and compression of sensor data and underwater images, the BLUENERGY project has the ambition to pose the scientific foundations for the development of automated, remote and real-time, monitoring systems enabling predictive and preventative operation and maintenance for the offshore renewable energy sector.
In our reference scenario underwater turbines are deployed in shallow water. Each turbine is instrumented with sensors, e.g., on the turbine blade, on the moorings, to monitor structural integrity, as well as with video-cameras.
Our objective is to study where to place the sensors, how to compress information and how to transmit it in real-time in presence of significant noise and fast varying channel conditions, to be able to provide the needed level of granularity of information required for risk assessment and forecasting, for system operation and maintenance optimisation. To achieve this challenging objective the project relies on a multidisciplinary team of internationally recognised researchers in three scientific area, coming from two different macro-areas (D and F), covering the field of sensing systems and networking, computer vision, multimedia and computer graphics.
The project will provide both fundamental results (algorithms, protocols) and experimental ones. The designed solutions will be validated through: a) testing of the effectiveness of the information compression and reconstruction techniques on datasets of underwater data and images; b) system simulations; c) small scale field experiments.
We discuss SoA and how we innovate in this section. Bibliography is included in the section "Eventuali altri partner esterni"
1) UNDERWATER COMMUNICATIONS AND NETWORKING
Networking protocols for UWSNs proposed so far attempt at addressing the unique features of underwater communications but fall short of successfully tackling the ever changing conditions of the network, including the varying traffic and, even more compelling, noise, link quality and general environmental settings.
Recent solutions for UWSN MAC and routing are surveyed in Li et al. [Li16] and more recently by Khan et al. [K18]. Among the many solutions, one that stands out in terms of overall remarkable performance is the Channel-aware Routing Protocol (CARP), exploiting link quality information for successful data delivery to the sink [B15]. Nodes are selected as relays based on their link quality, hop count and residual energy. The protocol MARLIN-Q presented in [B19] instead proposes a solution for quality-of-service based data delivery in multi-modal networks, namely, in networks whose nodes use multiple communication devices, such as acoustic modem and optical transceiver. The HYDRO protocol concerns single-route data forwarding in networks whose nodes are powered by different forms of energy harvesting [B18]. As such, the main concern of that routing strategy is to find routes of nodes with the smallest probability of running out of energy. Solutions presented in [Hu13], [Pla15] are also for networks with intermittent connectivity. A learning-informed routing protocol designed for UWSNs is QELAR, by Hu and Fei [Hu10]. Based on a model-based Q-learning approach, QELAR aims at maximizing the residual energy of the network nodes. Relays are chosen depending on the energy they can save by locally running a learning framework whose cost function accounts for the residual energy of each node as well as for the energy distribution among neighboring nodes.
INNOVATION WITH RESPECT TO SoA
In this project we will address the design of multi-frequency acoustic networks, designing an adaptive solution to learn and optimize based on channel conditions but also on noise level, a problem so far unaddressed which represents the requirements of the selected application scenario. We will also design network protocols jointly optimizing relay selection, coding and compression parameters.
2) IMAGE COMPRESSION, UNDERWATER IMAGE 3D RECONSTRUCTION AND MOSAICKING
Mosaicking process consists of the following main steps: feature extraction, feature matching, frame transformation, and, finally, image stitching. In [Li19] authors propose a method for underwater mosaicking of coral reef images acquired manually by scuba divers. Even if parallax and wave motions are in part addressed, issues about noises and artifacts show that the reference pipeline requires to be better investigated for underwater environments.
In [A17] authors propose sub-sampling techniques to obtain comprehensive maps, with low computational demand, starting from images acquired by robots. Image distortions, stitching time, and error propagation of current solution demand for further research to improve performance and quality.
Concerning anomaly detection [G12] proposes a method for detecting man-made objects in sonar images. Authors in [C17], instead, investigate the use of light information for detecting objects in an underwater environment. In particular, the global contrast of various features is used to initially identify the region of interest (ROI), which is then filtered by the image segmentation method. Despite the excellent results, the works reported use skillfully customized standard techniques to face an underwater problem like a non-underwater one, leaving wide room for improvement in terms of accuracy.
About the image compression task, in [R19] authors propose a real-time and progressive image compression method for ARM processor architecture based on the discrete wavelet transform. More investigations are required to further improve compression vs quality to cope with limited bandwidth available in UWSN.
Concerning underwater 3D reconstruction, authors in [B18] propose a method for recovering 3D information from inspection imagery. In detail, they present a novel stereo matching algorithm based on Markov random field and a pyramidal scheme. However, no specific solution tailored to underwater environment is available.
PROPOSED INNOVATION WRT SOA
In the project we will design a novel keypoint extractor and descriptor to support mosaicking pipeline as well as a novel raster-based background modelling to support novelty detection and compression problems. For the latter, we will develop an adaptive approach based on ROI concept to support high quality representation of object of interest. A third and final innovative contribution will regard adaptive geometrical-based background modelling to support synthetic 3D representations of underwater environments