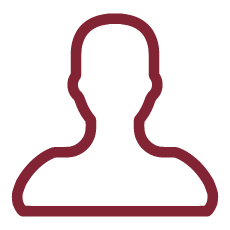
In the last few years, most of the western countries, alerted by the increasing of the populations and energy consumption with the consequent increment of fossil fuel emissions, started to promote and adopt several strategies aimed to an efficient, clean and sustainable production and utilization of the energy, especially in the transportation, the industrial and the domestic field.
One of the most relevant issue in this context is the efficient integration of the Renewable Energy Sources (RES) and Electric Vehicle (EV) public charge stations infrastructures that are both characterized by intermittent stochastic power profiles.
The spreading of such power systems, if are not intelligently managed, would increase the risk of outages, the waste of energy, local faults and accelerate the deterioration of electric infrastructure, in particular, the distribution grid.
Obviously all these circumstances are reflected on the cost of the dispatched kWh.
The need of an electric grid supported by an ICT infrastructure and distributed Energy Storage Systems (ESSs) able to manage such kind of energy production and assuring the power demand, the safety and stability of the electric grid is growing more and more.
Grid connected-Microgrids (MGs) are the most affordable solution for the development of smart grid infrastructures.
In this project it is proposed a novel approach based on graph theory in order to represent and manage the MG energy flows. It consists in focusing how much MG energy demand and energy production must be managed in real time respect with can be shifted or more in general controlled with a certain degree of flexibility in a given time horizon. Such model must be able to efficiently support the realization of a MG Energy Management System based on machine learning techniques and optimized through evolutionary algorithm therefore a data driven approach.
Furthermore, different tools and problem assumptions are need to formulate a suitable application scenario.
The adoption of machine learning can support the design phase and real time control strategy (i.e. MG EMS design) for this complex system affected by stochastic and non-linear dynamics, reducing the computational efforts as well. Fuzzy Logic, Neural Networks and Clustering techniques can be employed to design a robust, fast and efficient real time decision making system also applying a hybridization of these techniques (e.g. in literature are often mentioned as Adaptive Neural Fuzzy Inference System and Fuzzy K-means techniques) [1].
The utilization of fuzzy logic allow the design of a rule based system where the EMS way of thinking can be read and then interpreted [2].
Neural Networks (e.g. Recurrent Neural Networks or Echo State Networks) are widely used for the DERs power production prediction (i.e. wind turbine and solar PV). Also in this project the application of Neural Network will be investigated for the prediction of energy production from PV generation, MG loads and Fast Charge Demand as well. In particular, it will be investigated how the utilization of Neural Networks for the energy systems prediction must be efficiently applied in order to improve the overall EMS performance [3].
These systems once implemented in a specified problem can be optimized both off-line either online through a data driven approach that can rely for example on Evolutionary Algorithms such as Genetic Algorithm and Particle Swarm Optimization. These Evolutionary Algorithms can be applied on such kind of decision making inference systems in order to completely synthesised or efficiently tune their parameters even in case of multi objective optimization problems.
The EMS must be designed considering the minimization of MG operational costs without stress the connected power grid (i.e. a multi objective problem), namely reducing the power transients with the grids.
Deterministic methods like Mixed Integer Linear Programming and Dynamic Programming with the supporting of highly efficient energy systems predictors are widely mentioned in literature for these kind of problems. However, they require robust and highly efficient prediction algorithm and are affected by high computational costs per time slot. Moreover, their tuning can result extremely difficult in case of multi objective problems.
In this work such methods will be exploited for benchmark solution formulation in order to build and test the proposed EMS.
Once set the EMS, the investigation of 2^nd life battery from EV FS will be considered in order to observe additional MG operational costs reductions for supporting the installation of FC stations connected to the MG [4].
For these reason, it will be necessary implement a EV commuter load simulation tool in order to formulate a proper scenario in which the FC station is supposed to be installed. This tool must simulate typical EV commuters¿ trip in a given area where the MG and the FC station are supposed to be installed [5]. This toolbox must be able to provide useful information for generating a dataset that represents a typical FC station load over an extended period of time in order to train and test MG EMS.
A graph model will be developed to represent and study the MG energy flows and the EMS performances, respectively.
The installation of a controlled conventional generator for supporting the MG auto consumption and reducing the energy oscillation between MG and the distribution grid and the overall costs will be investigated too.
[1] R. Deng, Z. Yang, M. Y. Chow and J. Chen, "A Survey on Demand Response in Smart Grids: Mathematical Models and Approaches," in IEEE Transactions on Industrial Informatics, June 2015.
[2] S. Leonori and E. De Santis and A. Rizzi and F. M. F. Mascioli, IEEE Congress on Evolutionary Computation (CEC), ¿Multi objective optimization of a fuzzy logic controller for energy management in microgrids¿, July 2016.
[3] Stefano Leonori and Alessio Martino and Antonello Rizzi and Fabio Massimo Frattale Mascioli, IJCCI, ¿ANFIS Synthesis by Clustering for Microgrids EMS Design¿, 2017.
[4] Ellingsen, Linda Ager-Wick and Singh, Bhawna and Stromman, Anders Hammer, Environmental Research Letters, ¿The size and range effect: lifecycle greenhouse gas emissions of electric vehicles¿, 2016.
[5] G. Celli, G. G. Soma, F. Pilo, F. Lacu, S. Mocci and N. Natale, "Aggregated electric vehicles load profiles with fast charging stations," 2014 Power Systems Computation Conference, Wroclaw, 2014.