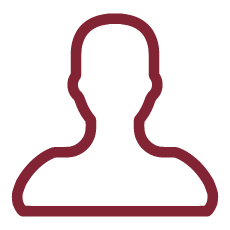
Machine Learning methods have changed the approach to statistical analysis in many fields. In this project we propose to apply and fit the latest technologies in adversarial machine learning to the solution of turbomachinery flows, and in particular to three challenges that are still opened in this field: generation of syntethic turbulence for LES inflow conditions, turbulence modelling in rotating passages, particle cloud tracking.
To this aim an open-source platform will be created, with tools for Exploratory Data Analysis, to reduce and select the significant features in each problem.
Then, adversarial machine learning and variational auto encoders will be exploited to generate a synthetic turbulence for LES inflow conditions. In this way we expect to be able to train an artificial neural network to correctly reproduce the inherent statistics of turbulence. Another work package will be dedicated to correct RANS modelling of rotating passages using a machine-learnt model able to account for the effects of rotation and correct a standard model using LES or DNS data.
The third problem to study will be the use AI to predict the statistical behaviour of a cloud of particles dispersed inside a turbomachinery flow.
All the work will be carried out using our in-house CFD codes that will be interfaced with a Python platform of machine learning techniques through the C++ APIs for Python bridging.
The key innovation aspects in this research are the development of machine-learnt strategies for TM flows, based on the latest advancement of ML.
When dealing with EDA, the possibilities of adapting statistical methods were already demonstrated in two papers recently published by our research group. In the work we are currently carrying out EDA techniques were tuned to handle TM problems and TM flows, formulating new normalization strategies that better perform when dealing with data from numerical computations, and ML algorithms for feature selection and reduction were successively used for TM design.
With respect to active ML techniques, adversarial ML and the use of VAEs have been introduced in the latest 4 years in statistical science and their application in TM flows modelling is expected to solve a number of limitations we had so far.
Still today, the modelling of inflow conditions in LES is an open problem that so far no analytical formulation was able to tackle. The intrinsic non-linearity of ANNs is considered as a possible remedy to this and the concept of adversarial networks provides a secure instrument to check the accuracy of the algorithm. Being an evolution of adversarial ANNs, VAEs can be seen as a possible alternative. Finally the application of both methods in this field would be completely innovative per se, as at the knowledge of the PI, so far no application to turbulence modelling is present.
At the same time, the problem of handling rotating frames of motions and the deformation of secondary motions in rotating passages was never explored before with ML techniques (the only exception being a work by Ling et al, that was limited to non-rotating passages). A corrected RANS model for rotating frame of reference and secondary motions would increase the accuracy of RANS models in many cases of radial turbomachinery, that is still an open issue especially when dealing with centrifugal pumps and fans with very narrow passages (i.e. very low blade axial span with respect to external radius 1-5mm vs 50-500 cm).