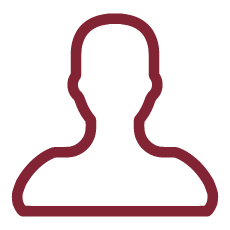
In performing a statistical analysis, one of the most fundamental steps is related to the choice of the sample size. In general, the sample size determination (SSD) problem deals with the selection of the optimal number of subjects to be enrolled in a study in order to achieve a pre-specified inferential target. In the literature this problem has been addressed from both a frequentist and a Bayesian point of view (and anything in between), but often the Bayesian paradigm is preferred since it is possible to blend and balance the strength of the (soon to be) observed empirical evidence with all the prior knowledge already available. We decide to focus on the case of a "community of priors" representing, for example, multiple scenarios we want to account for, or even different expert opinions. Broadly speaking, within this setup, our SSD effort is directed toward the selection of the smallest sample size that guarantees "agreement" between these, possibly conflicting, prior opinions. We formalize the loose idea of "agreement" in terms of the Wasserstein distance between posterior distributions stemming from different priors, and we show how to approximate this quantity exploiting some recent results based on Stein's method. We detail our procedure in the case of the conjugate Binomial model -- due to its importance in the context of clinical trials -- and also of the ubiquitous conjugate Normal model, comparing our optimal sample sizes with those provided by more standard techniques.
As we have already pointed out, in a Bayesian framework, our sample size determination effort is directed toward the selection of the smallest sample size that guarantees "agreement" between possibly conflicting expert opinions.
To the best of our knowledge, the use of the Wasserstein metric to deal with the selection of optimal sample size is novel in literature. In our research we think to be able to carry relevant contributions in both methodological and computational fields.
Expected contributions which are innovative with respect to the state of the art are:
1) MULTIPLE COMPARISONS: as of now, the Wasserstein distance can be computed only with respect to two probability distributions. Since we deal with several posterior distributions -- stemmed from several expert opinions -- we think to build up a specific algorithm which iteratively computes Wasserstein distances between all the considered distributions. At the end we arrive with an overall Wasserstein distance between these distributions which can be used as starting point for the evaluation of Bayesian sample size criteria;
2) NEUTRAL DISTRIBUTION: as said before, the Wasserstein barycenter is useful to summarize, in an efficient and understandable way, several probability distributions. In the context of equivalence experiments framework, the main aim is to assess statistically when a new advantageous treatment is clinically equivalent to its older competitor. A major role -- in this particular problem -- is played by the so called neutral probability distribution, which is assigned once the neutrality interval is obtained. Using the Wasserstein barycenter we could remove the arbitrariness on the choice of neutral distribution and, in this sense, the barycenter can be viewed as a novel method for obtaining neutrality distribution;
3) THRESHOLD SELECTION: another important aspect, strictly related with the optimal sample size, deals with the thresholds used in Bayesian criteria. In general, wrong thresholds can lead to miss-specified optimization problem and hence we need to choose them carefully. In terms of the Wasserstein version of the Bayesian criteria, we propose specific methods for finding such thresholds in order to always obtain the requested optimal sample size;
4) ROBUSTNESS: many authors have focused on the problem of the sample size determination from a robust point of view, both in a simple and in an equivalence trials frame work, see again [1, 2]. In our research we think to set up the problem of robustness in terms of Wasserstein discrepancy.
Main references:
[1] S. Gubbiotti and F. De Santis, A Bayesian method for the choice of the sample size in equivalence trials, Aust. N. Z. J. Stat. (2011).
[2] P. Brutti, F. De Santis and S. Gubbiotti, Bayesian-frequentist sample size determination: a game of two priors, Metron (2014).