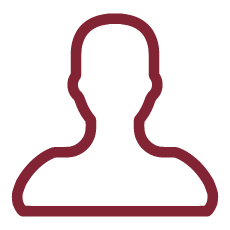
Within a Bayesian framework, the main goal of this project is to systematically investigate the use of optimal transportation methods in the design of statistical experiments, with a particular emphasis towards applications to sample size determination and planning of (possibly) high-dimensional clinical trials.
Optimal transport (OT) distances between probability measures in general, and the family of Wasserstein distances more in particular, have a long and well established history in probability theory. In more recent years, they have also found their way into statistical theory, applications and machine learning, not only as a theoretical tool but also as a quantity of interest in its own right. A non-exhaustive list of examples include goodness-of-fit, two-sample and equivalence testing; classification and clustering; exploratory data analysis via Frechet means and geodesics in the Wasserstein metric.
Despite this overflow of interest in OT, as today, its use and usefulness in the broad area of statistical experimental design seems to be only marginally explored.
Experimental design involves the specification of all aspects of an experiment, and decisions must be taken before data collection, usually under resource constraints. For this reason, at the design level, it is crucial to efficiently exploit all the relevant information available prior to experimentation, making Bayesian methods central.
Historically, the decision theoretic approach to (Bayesian) experimental design has been dominated by information criteria like Fisher information metric and Kullback-Leibler divergence, but recent developments suggest that, if we are willing to pay a small computational overhead, we can switch to the OT framework inheriting its robustness, shape preservation property and sensitivity to the underlying geometry without losing the original interpretability.
An extensive exploration of this idea in a variety of specific contexts is the leading theme of our proposal.
The participants of this project have a consolidate and multi-year experience in the area of experimental design and in the methodological research related to experimental design and clinical trials. Hence, we expect to provide innovative contributions from a methodological point of view in relation to all the aforementioned tasks that schematically outline the main objectives of our proposal.
REFERENCES
+ Bernardo (1979). Expected information as expected utility. The Annals of Statistics.
+ Blanchet, Murthy and Si (2019). Confidence Regions in Wasserstein Distributionally Robust Estimation. https://arxiv.org/abs/1906.01614
+ Box (1982). Choice of response surface design and alphabetic optimality. Utilitas Math. B.
+ Brutti and De Santis (2008). Robust Bayesian sample size determination for avoiding the range of equivalence in clinical trials. J Stat Plan Inference.
+ Brutti, De Santis and Gubbiotti (2008). Robust Bayesian sample size determination in clinical trials. Stat. Med.
+ Brutti, De Santis, Gubbiotti (2009). Mixtures of prior distributions for predictive Bayesian sample size calculations in clinical trials. Stat. Med.
+ Brutti, De Santis and Gubbiotti (2013). Robust bayesian monitoring of sequential trials. Metron.
+ Cuturi and Doucet (2014). Fast Computation of Wasserstein Barycenters. Proceedings of the 31st International Conference on Machine Learning.
+ De Santis (2006). Sample size determination for robust Bayesian analysis. J. Am. Stat. Assoc.
+ De Santis and Gubbiotti (2017). A decision-theoretic approach to sample size determination under several priors. In: Applied Stochastic Models in Business and Industry, John Wiley & Sons Ltd.
+ De Santis and Gubbiotti (2018). A predictive measure of the additional loss of a non-optimal action under multiple priors. In: Book of short Papers SIS 2018.
+ Etzioni and Kadane (1993). Optimal experimental design for another's analysis. J. Am. Stat. Assoc.
+ Gao et al (2018). Robust Hypothesis Testing Using Wasserstein Uncertainty Sets. NeurIPS 2018, Montréal, Canada.
+ Ghaderinezhad and Ley (2019). Quantification of the impact of priors in Bayesian statistics via Stein's Method. Statistics & Probability Letters.
+ Gneiting and Raftery (2007). Strictly Proper Scoring Rules, Prediction, and Estimation. J. Am. Stat. Assoc.
+ Gubbiotti and De Santis (2011). A Bayesian method for the choice of the sample size in equivalence trials. Aust. N. Z. J. Stat.
+ Hyvarinen (2005). Estimation of Non-Normalized Statistical Models by Score Matching. Journal of Machine Learning Research.
+ Joseph and Belisle (2019). Bayesian Consensus-Based Sample Size Criteria for Binomial Proportions. Stat. Med. (accepted)
+ Jackson, Novick, and DeKeyrel (1980). Adversary Preposterior Analysis for Simple Parametric Models. In: Bayesian Analysis in Econometrics and Statistics, North-Holland.
+ Lindley (1972). Bayesian Statistics - A Review. SIAM.
+ Lindley (1997). The choice of sample size. The Statistician.
+ Lindley and Singpurwalla (1991). On the Evidence Needed to Reach Agreed Action Between Adversaries, With Application to Acceptance Sampling. J. Am. Stat. Assoc.
+ Overstall and Woods (2017). Bayesian design of experiments using approximate coordinate exchange. Technometrics.
+ Sambucini (2019). Bayesian predictive monitoring with bivariate binary outcomes in phase II clinical trials. Computational Statistics and Data Analysis.
+ Thorarinsdottir, Gneiting and Gissibl (2013). Using Proper Divergence Functions to Evaluate Climate Models. SIAM/ASA J. Uncertainty Quantification.
+ Walker (2016). Bayesian information in an experiment and the Fisher information distance. Statistics & Probability Letters.
+ Weerahandi and Zidek (1981). Multi-bayesian statistical decision theory. J. R. Stat. Soc. Ser. A.