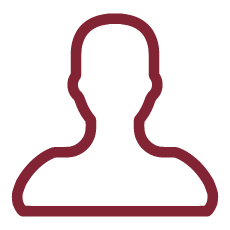
In this project, we propose an optoelectronic implementation of a Long Short-Term Memory (LSTM) layer. The optoelectronic technology is adopted in order to implement LSTM units in a stacked recurrent deep neural network. For the latter, we aim at introducing for the first time full analog computations that also cope with low-power constraints. The proposed system is based on standard microelectronic technology, while discrete-time delays are achieved via an optical line based on a long single mode optical fiber spool, lighted by a laser source tuned by a Mach-Zehnder modulator. This set-up allows a delay in the order of nanoseconds and adjustable by means of adapting the MZM parameters, thus there is no need for discrete quantization. Moreover, the low-power consumption, as well as the ability to perform computations with no finite precision and numerical constrains, are two prized features for embedded systems and smart sensors in the era of big data and, more in general, of real-world applications dealing with multiple data sources (i.e., IoT, Smart Grids, Intelligent Transport Systems, environmental control, home automation, e-health, and so forth). By the achievements obtained in the R&D activities and experimental assessments of this project, we will offer the opportunity to evaluate pros and cons of analog optoelectronic implementations, with respect to numerical approaches based on standard C-MOS technology, in terms of numerical performance, precision, power consumption, scalability, replicability, economical cost. Since, to our knowledge, there are no analog implementations of LSTM cells for Deep Learning proposed so far, either relying on optoelectronic technology, as in this project, or using microelectronic integrated components, the expected results may achieve an advancement of knowledge compared to the state of the art. Hence, the main goal of this project is to suggest a novel discrete-time analog implementation of recurrent deep neural networks.
Fast computation is getting a crucial issue in several applications where constraints on limited hardware resources might be imposed. Recently, low-power and cheap devices have started to use deep neural networks and, more in general, machine learning techniques to solve real-world data processing problems. Internet of Things (IoT), cloud computing, pervasive computing and so on, have revolutionized the way signals are processed and information is managed. To infer knowledge from big data partitioned over geographically distinct locations is considered a fundamental problem in many scientific fields now, including sensor networks, smart grids, pollution control, medical applications and many others.
In those cases, the problem consists in forecasting the future values of time series, which are related to some observed and measured physical parameters. This implies the adoption of dynamical data-driven models for obtaining a suited prediction accuracy, therefore relying on recurrent neural networks either based on shallow architectures (i.e., ESNs and, more in general, RC) or stacked deep models based of several recurrent layers (i.e., LSTM, GRU, etc.).
As a consequence of this choice, the adopted models are characterized by a further complexity that, on one hand, means more hardware units, more binary C-MOS commutations and hence, more power consumption. On the other hand, setting a limit to those parameters means to reduce the hardware complexity, which in turn yields digital architectures with fewer bits and a reduced numerical precision. Moreover, in this kind of scenario, it is often forbidden to transmit all data to a centralized authority, for reasons of security, privacy, computational efficiency and economical costs. Computation is constrained to be performed in a network of interconnected computing agents, where each agent is usually characterized by a low-cost device, usually embedded into a smart sensor, which often must save energy for a long battery autonomy.
Overall, these approaches are often computationally intensive and memory demanding, making difficult to implement them on a simple hardware like a microcontroller. Nonetheless, the parameters are estimated via learning algorithms running on standard computers with double precision floating point arithmetic. However, uploading the network model on a digital architecture with finite precision arithmetic, after a direct quantization of coefficients, leads to unsatisfactory results due to the nonlinear nature of the network. In addition, many real-time applications need an adaptive learning, as in the case of the well-known consensus strategy, where the model must be dynamically adapted to new observations even after the hardware implementation.
So, the main goal of this project is to propose a sound basis for the discrete-time analog implementation of deep neural networks, so as to carry on a detailed investigation about costs and benefits associated with the analog hardware with respect to a numeric implementation based on C-MOS technology. The main problem in this regard consists in the implementation of the delay line in order to control synchronously the data processing flow. To this end, we are proposing an optoelectronic implementation where the delay is obtained in the optic domain, therefore with a granularity of nanoseconds that is fully compliant with the minimum clock period determined by the group delay of the microelectronic analogue processing.
Although the literature is plenty of different proposals regarding the optoelectronic implementation of ESNs as well as shallow neural networks based on the RC paradigm, to our knowledge, there are no analog implementations of LSTM cells for Deep Learning, either based on the optoelectronic technology, as in the present project, or even using microelectronic integrated components. Consequently, apart from the innovative approach regarding a first and novel hardware implementation of a deep cell, it is evident that we may achieve an advancement of knowledge compared to the state of the art in the literature also taking into account the experimental results that are expected to be obtained. Namely, they regard the comparison in terms of speed, power consumptions and numerical accuracy of the proposed approach with respect to the number of bits adopted in standard digital implementations and the related fixed or floating point numerical precision. Consequently, we expect the scientific community to benefit of the results obtained in this study, so as to overcome the above-mentioned issues, which actually prevent a broader diffusion of deep learning technologies in smart sensor networks, which are increasingly adopted in real-world engineering applications nowadays.