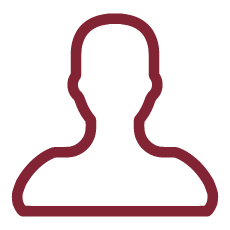
We study the penalised complexity priors and their applications in a Bayesian framework. For instance, some of the applications we are working on are related to the elicitation of the prior distribution for the shape parameter of univariate skew symmetric models; other applications concern copula modelling. In our project, we make use of a new concept for constructing prior distributions. We exploit the natural nested structure inherent to many model components, which defines the model component to be a flexible extension of a base model. These proper penalising complexity priors are defined to penalise the complexity induced by deviating from the simpler base model and are formulated after the input of a user-defined scaling parameter for that model component, both in the univariate and the multivariate case. These priors are invariant to reparameterisation, have a natural connection to Jeffreys' prior, are designed to support Occam's razor and seem to have excellent robustness properties, all which are highly desirable.
One of the principal innovation traits of this research is the possibility to derive a prior distribution in every context in which a model can be seen as a flexible version of a simpler base model. From a Bayesian point of view, non-informative (or weakly informative) priors avoids subjectivity and are very popular in the recent literature. Another important aspect is that the user can define an upper bound that specifies what we think about a tail event and the probability we put on this event. This condition allow the user to prescribe how informative the resulting Penalised Complexity prior is.
Another important advantage of the PC priors is the robustness. Since it is well-known that in the hierachical models there is the drawback to shrink too much towards zero, robust distributions are needed.
With regard to the copulas, a fundamental result we got is the fact that the Penalised Complexity prior for the copula parameter does not depend on the marginal densities we use to construct the joint density. In other words, what is relevant to find the PC prior for the copula parameter is just the copula structure, regardless the marginal distributions involved. The innovation, in this case, is that we can construct PC priors for different copulas avoiding the information on tha marginal densities.