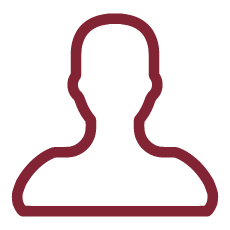
Social media radically changed the way we access information and form our opinions. Users online tend to acquire information adhering to their beliefs and ignore dissenting information ([1, 2]). Such a process, combined with the unprecedented amount of information we can access online, fostered the emergence of groups of like-minded peers framing and reinforcing a shared narrative (i.e., echo chambers). The exceptional and unprecedented global effects generated by the COVID-19 epidemic have shown how social media can be an effective tool for influencing population behavior, helping governments in epidemic management. The COVID-19 pandemic elicited the limits of the definition of "Fake News" in capturing the overall complexity of the new information landscape. Indeed, the World Health Organization coined the term infodemics to define the "overabundance of information - some accurate and some not - that occurs during an epidemic." The typical approaches for countering misinformation include, among the others, improving detection algorithms, introducing legal deterrents, developing a more educated online citizenship. However, misinformation campaigns are still considered effective and still difficult to prevent.
The Prebunking project aims to tackle this important challenge, studying the state of the art of the so-called Coordinated Inauthentic Behaviors (CIBs), evaluating their characteristics, and proposing possible countermeasures to limit their impact. In particular, leveraging the experience of the proponents about the topic, we will:
- search for effective modeling of CIB attack strategies that aim at promoting ("making viral") selected contents, subverting social media platforms feed algorithms;
- propose possible countermeasures to reduce the effects of CIB attacks, and thus limiting the effectiveness of misinformation campaigns;
- develop specific approaches and more generalized strategies to limit as soon as possible the effects of misinformation spreading.
The Prebunking project aims at finding novel tools for battling disinformation spreading, as an effective complementary measure to debunking and fact-checking. In particular, the project will bring:
1) innovative modeling of CIBs that combine together behavioral features and content features;
2) early warning system for CIBs, made of tools able to identify possible signs of CIB activities at their early stages;
3) possible countermeasures to efficiently impact the effects of CIB campaigns;
4) implementation of a proof of concept prototypal system that integrates the above components.
Innovative modeling of CIBs
We defined CIB as those actions that try to interfere with the feed algorithms of social platforms to promote some (possibly fake or polarized) content to go viral. Thus, CIBs generally combine actions of bots, fake and hijacked accounts, people with extreme opinions or legitimate users, possibly victims of some form of misinformation [28, 29, 30]. We observe that, in the academic literature, those kinds of actors have received great attention, but still, a unifying model is missing. We plan to fill this gap and to provide generalized modeling of CIBs actors, also combining content-based features, considering that fake news and fake media detection techniques have proved to be quite effective [12, 13]. We think that our model will be a reference for several other phenomena on social media platforms.
Early warning system for CIBs
Since debunking activity happens after the spreading of misinformation, it likely happens after negative effects have germinated in the community. We think that a system able to raise early warnings and identify CIBs at their very early stages can be a powerful tool in the hands of both citizens and media experts. For example, we envision our system as a public website or an app for the citizens, useful for predicting the future CIBs' topics or targets. Moreover, given our generalized approach, we imagine the system as a component for Governative agencies' tools, where additional features can be combined to further improve the prediction quality. It is acknowledged that Governative agencies actively work and collaborate with social platforms to fight disinformation [31].
Countermeasures against CIBs
We think that the "prebunking" concept is very promising for fighting disinformation. However, in the literature, it has been only used as an implementation of the inoculation theory, for providing users with some immunization against misinformation [18]. With the Prebunking project, we plan to go beyond this literal meaning and we plan to bring new tools to make CIBs campaigns less effective. For instance, we expect to build tools to secure multimedia content, such as videos, augmenting them with "adversarial noise" that prevents them from being manipulated. Our innovative claim is that the adversarial noise [32] can be used to disrupt the creation of DeepFakes [33], thus working proactively to preserve and defend personal users' content, and preventing CIBs upstream.
Prototype Implementation
To have a method that can be usable, we need to consider that it needs to process a huge amount of data. We will tackle this processing using innovative algorithms and designing a proof of concept implementation oriented to high-speed data processing. In particular, we will use streaming algorithms for the collection of features/metrics. Focusing on the use of hash-based approximate data structures (Counting Bloom filters, HyperLogLog sketches, min-hash, and local sensitive hashes [34]), and sampling techniques, we will design efficient software primitives able to process the huge amount of data generated by social media, beyond the state-of-the-art. Among the results, we will bring a set of scalable and programmable data structures able to provide and process the features of our modeling. We envision that our proposed data structures will be general enough to be adapted and used to face other social media problems.
Further references
[28] Cresci S., Di Pietro R., Petrocchi M., Spognardi A., Tesconi M., Fame for sale: Efficient detection of fake Twitter followers, Decision Support Systems, 2015 (80)
[29] Egele M. et al., Towards Detecting Compromised Accounts on Social Networks, IEEE Transactions on Dependable and Secure Computing 2017, 14(4)
[30] Xia Y. et al., Disinformation, performed: Self-presentation of a Russian IRA account on Twitter. Inf. Commun. Soc. 2019 (22)
[31] https://www.washingtonpost.com/technology/2020/09/01/facebook-disinforma...
[32] Madry A. et al., Towards deep learning models resistant to adversarial attacks, ICLR 2017
[33] Ruiz N. et al., Disrupting Deepfakes: Adversarial Attacks Against Conditional Image Translation Networks and Facial Manipulation Systems, ECCV Workshops 2020
[34] Bruschi V., Reviriego P., Pontarelli S., Ting D., Bianchi G., More Accurate Streaming Cardinality Estimation With Vectorized Counters, IEEE Networking Letters, 2021 3(2)