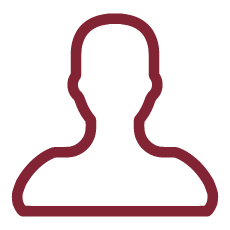
Patients affected by chronic diseases need frequent outpatient visits for assessing the disease and symptoms evolution and the therapy. However, having a complete medical case is not such a trivial task since it is often based on the patient's subjective feelings, making a focused treatment not feasible. Using remote long-time monitoring in domestic environment can represent an efficient and valid alternative to patient commuting and hospitalization. In this frame, wearable sensor systems allow the implementation of telemedicine strategies with the aim of providing an objective and complete overview of the patient's health by recording specific physical and physiological parameters associated to the disease during the whole day and in free-living-conditions. In Parkinson's Disease (PD), an uncertain comprehension of patient's condition can lead to a wrong medicine dose and to complications which can determine the manifestation of several disturbs, such as the Freezing of Gait (FOG). FOG is a very common and risky symptom of PD, which often leads to catastrophic injuries and falls. Starting from the scientific collaboration between the research group of Prof. Irrera and some Neurologists of the Sapienza Department of Human Neuroscience on the specific topic of the automatic recognition of FOG, We propose here to use a wearable system including inertial sensors and surface Electromyography (sEMG) to predict the FOG occurrence in order to alert the patient and prevent falls. The challenge is to find a specific correlation between characteristic patterns of the leg muscle action potentials and inertial signals which are typical of a condition immediately preceding the FOG manifestation. The entire analysis will be performed in a low-power and stand-alone wearable system, able to monitor the patient in a free-living environment. The achievement of this target would be an important step toward the reduction of the patient fall risk and the improvement of the life quality.
Wearable solutions which implement FOG predicition are usually based on sensors attached to various parts of the body, which communicate with an external unit, i.e. a PC or a smartphone. The role of this unit is to receive data from the sensors and to elaborate them in order to classify the different gait conditions, i.e regular gait, rest, pre-FOG and FOG. The presence of an external unit impacts on the comfort and on the battery life of the entire system, since every sensor has to send data to the external unit. Furthermore, these solutions consider solely one kind of sensor, either inertial, or sEMG, or ECG, or SC, or EEG, achieving a percentage of FOG events predicted up to about 71%.
The purpose of the present proposal is to assess whether the occurrence of FOG events could be predicted on the basis of the presence of specific patterns in both inertial and sEMG signals just before a FOG manifestation. The reason why we want to use several signals rather than one is due to the robustness of the pre-FOG case classification. Indeed, FOG can be preceded by typical muscular and movement adjustements, such as the increased TA activity and a degradation of the gait pattern [1, 2]. So, by comparing or fusing the two signals, the FOG prediction reliability would be more likely to increase, going beyond the 71% obtained so far. Furthermore, as reported in [3], the use of both sEMG and inertial signals allows to perform a classification between trembling in place FOG and shuffling forward FOG. In this way, it can be possible to study the pre-FOG event characteristics depending on the FOG phenotype, thus evaluating if different patterns are present in the pre-FOG phase of the two phenotypes. The sub-classification of the two pre-FOG types and their inspection can be useful in order to extend the knowledge of the mechanisms prior to a FOG event and to define a different relevance level of the risk of fall depending on the FOG phenotype. The complete overview of the FOG characteristics in all its temporal stages (pre-FOG and FOG) so obtained can be employed to implement a custom feedback solution for decreasing the occurrence of the subsequent FOG manifestation. This solution can then be different depending on the FOG phenotype, whether in the nature of the feedback (uditive, visual, etc) or in its characteristics (frequency, amplitude, etc). In addition, associating a relevance level to the fall risk would permit to activate the feedback just in case of necessity, since an over-stimulation of the patient can lead to an intensification of the FOG manifestation instead of its reduction. Finally, monitoring the patient all day long, it is possible to appreciate the efficacy of the various feedback solutions on the unfreeze mechanism, i.e. the FOG event overcome, and to choose the appropriate one for the single patient.
In conclusion, the proposed research project aims to offer a wearable system which can extend the knowledge of the FOG and pre-FOG mechanisms, improves the FOG prediction and implement the best solution for decrease the FOG manifestations and the risk of fall. This can bring benefits in terms of patients and caregivers' quality of life and of cost reduction for the National Health Service.
[1] A. Nieuwboer, R. Dom, W. De Weerdt, K. Desloovere, L. Janssens, and V. Stijn, "Electromyographic profiles of gait prior to onset of freezing episodes in patients with Parkinson's disease", Brain, vol. 127, no. 7, pp. 1650-1660, 2004.
[2] L. Palmerini, L. Rocchi, S. Mazilu, E. Gazit, J. M. Hausdorff, and L. Chiari, "Identification of characteristic motor patterns preceding freezing of gait in Parkinson's disease using wearable sensors", Front. Neurol., vol. 8, no. AUG, pp. 1-12, 2017.
[3] I. Mazzetta, A. Zampogna, A. Suppa, A. Gumiero, M. Pessione, and F. Irrera, "Wearable Sensors System for an Improved Analysis of Freezing of Gait in Parkinson's Disease Using Electromyography and Inertial Signals", Sensors (Basel)., vol. 19, no. 4, 2019.