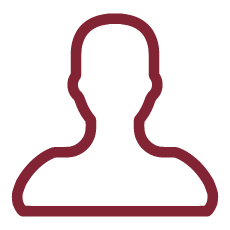
The present project proposal is part of a larger project that aims to implement maintenance strategies based on condition (condition-based maintenance) applied in the industrial sector. Consistently with the current national and production developments, the increasing attention to the acquisition of the process data at the machines, we want to build a model based on machine learning techniques to automatically identify the process phases of an industrial machinery, as well as highlight any abnormal behavior. In fact, several production systems/machine performs different processing phases and the process data are not automatically linked to the specific activity performed. The proposal stems from the industrial needs repeatedly collected by the research team, both by machine builders and users. As an example, the pharmaceutical production, particularly present in the Lazio with interest of Sapienza University, is based on production processes of granulation of the powders; this process is carried out inside a single machine that deals with preheating, conditioning, components addition, product rest, steam, etc., but collected data of the machine (e.g. outlet air temperature, or product humidity, etc.) has not the link to specific process phase. It should be emphasized in such contexts, that a predictive condition maintenance system leads to important cost reductions, avoiding production downtime or product loss, and minimizing the frequency of periodic maintenance interventions. The present research aims to use machine data available in the research group, acquired from real industrial contexts, to develop a machine learning system able to identify phases and anomalies, and a measure of criticality of the observed situation.
As seen in the previous paragraph of the proposal, currently effective data labeling tools are not available for complex production processes, with many phases and parameters. The research will study several machine learning algorithms and it will develop comparison tool as a model to identify the best solution to identify production phases by collected data. The innovation of the research will also be the linking of this labeling activity to other anomalies identification tools, as will be selected in the literature. New insights into the specific process focused in the research will help producer and machine vendors to get information about the best maintenance policy and needs, with important managerial support. The main challenges that the research will overcome promoting an advance of knowledge are the possibility to deals with data noise, which might be similar to abnormal behavior because the boundary between normal and abnormal behavior is often not precise. Moreover, the implementation of a machine learning model will provide a new innovative way to manage cases where the definition of abnormal or normal may frequently change, e.g. for different production scenario (different products). Lastly, the main advance considering the state of the art in maintenance improvements with machine learning will be the capability of the model to recognize phases through patterns, with more sophisticated methods, such as decomposing the data into multiple trends in order to identify the changes in activities.
All the modelling will be implemented using Python to provide freely available modules for further researches.