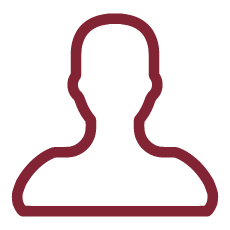
The use of Quantile Regression to estimate the conditional quantile of the total claim amount for car insurance policies was originally considered by Kudryavtsev (2009). In this paper, we first propose to use the well known Quantile Regression Neural Networks (QRNN) procedure introduced by Taylor (2000) in an insurance ratemaking framework which has never been proposed before. Secondly we extend the Combined Actuarial Neural Network (CANN), proposed by Wuthrich and Schelldorfer (2019), to a quantile regression contest building a Quantile-CANN model combining the traditional Quantile Regression approach with a Quantile Regression Neural Network to estimate the conditional quantile of the total claim amount.
We adopt a two part model approach fitting a logistic regression to estimate the probability of positive claims and both QRNN and the Quantile-CANN models for the the positive outcomes. Last but not least we use the estimated quantiles to calculate a loaded premium following the Quantile Premium Principle considered in Heras et al. (2018).
This research project aims at introducing machine learning techniques in the context of quantile insurance pricing. Nowadays, the issue of quantile claim amount estimation is addressed by means of tradional regression techniques (i.e. Quantile Regression). Our proposal is to evolve this approach introducing Neural Networks models in order to achieve a greater precision in the estimation of the quantile of the total claim amount. To this end we propose two techniques: QRNNs, that have already found application in other fields, but that have yet to applied in actuarial sciences; Quantile-CANN is a new extension of the CANN approach devoted to conditional quantile estimation, that combines Quantile Regression and QRNNs.