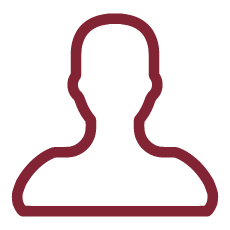
Background
Breast magnetic resonance imaging (MRI) is of fundamental importance in breast cancer care nowadays, having widely recognized indications, from locoregional staging to the evaluation of the response to NAC, and more recently has been employed to investigate biomarker information. Radiogenomics refers to the integration of image-extracted phenotypes and genomic data of the same tumor, which has the potential to improve both diagnosis and therapeutic strategies for evaluating the individualized disease signatures with higher accuracy. MicroRNAs (miRNAs), which are short (18-22 nucleotides) non-coding RNA sequences, are novel biomarkers of breast tumorigenesis with the ability to regulate hundreds of genes and biological pathways. They are appealing targets for screening, diagnosis, prognosis and for the choice of correct treatment.
Hypothesis
Our purpose is a computational approach of breast cancer (BC), that correlates phenotypes from magnetic resonance imaging (MRI) with genotypes and miRNAs expression, developing a radiomiRNomic map. In order to discover the relationships between MRI texture analysis and miRNA expression data derived, the radiogenomic strategy could open new frontiers in Breast Cancer Precision Diagnosis and Prognosis.
Aims
The project has two aims:
1.precision lesion assessment and diagnosis. To noninvasively predict the molecular subtype of BCs, attributing luminal A, luminal B HER+, luminal B HER-, non-luminal HER2+, or triple-negative/basal-like.
2.precision diagnosis and prognosis. To noninvasively correlate radiomics information with molecular subtype of BCs and miRNAs profiling.
Expected Results
1.To noninvasive predict molecular BC subtypes by an artificial intelligence-based algorithm of radiomics features.
2.To identify new radiomiRNomic profiles of multi-omics biomarkers, which have the potential to improve both diagnosis and prognosis with the ultimate aim of personalizing therapeutic strategies.
In the last decade, researches have demonstrated that distinct tumor phenotypes can show specific imaging texture features [1]. For this reason, new perspective of breast MRI imaging cannot ignore radiomics (which use algorithms of texture analysis to extract large volumes of quantitative imaging data and integrate them with clinical information) and radiogenomis (that is the combination of genetic and radiomic data), whose final purpose is to create non-invasive biomarkers able to predict breast cancer risk and outcomes, based on MRI signatures from high-throughput platforms. In particular, recently several Authors have suggested that radiogenomic markers can successfully distinguish among molecular breast cancer subtypes [2,3] and can identify a priori unresponsive patients among those candidates for neoadjuvant therapies (NAT). In this perspective, several studies [4,5] have evaluated the potential of applying radiomics signatures and machine learning classifiers to Multiparametric-MRI (MP-MRI) to predict who will benefit from NAT. In addiction, recently some Authors [6] have proposed to use deep learning methods to predict response to NAT using pre-treatment MRI only. Despite these promising results, the use of machine learning applied to MP-MRI of the breast to distinguish among molecular breast cancer subtypes and for early prediction of response to NAC is still investigational. Relatively small and retrospective studies were performed so far, limiting the statistical power and the generalizability of those results which represent a first step in the long way to develop imaging biomarkers as a surrogate for gene profiling. In this scenario, miRNAs are emerging as interesting minimally invasive biomarkers for several tumors [7-9]. Global miRNA expression studies have identified miRNAs consistently dysregulated across many types of cancer, and other dysregulated miRNAs more specifically associated with a particular cell type or organ site of cancer, as of potential clinical relevance. We expect to perform a global miRNA expression microarray study on a series of BC grouped by stage, grade and molecular subtype, in order to identify miRNAs putatively involved in the biology of BC diagnosis and prognosis. MiRNAs identified as potential biomarkers will be studied in our cohort of patient using ddPCR that allows absolute quantification. Moreover, we expect to identify signatures of circulating (ev-associated) miRNAs that can be integrated with imaging texture features in the diagnosis and management of BC patients in a minimally invasive way. Thus, the final aim of our study is that the precision radiogenomic tool allows an evaluation of the tumor biology independent from that obtained from the needle biopsy and that it correlates the radiogenomic information with the miRNA profile, to offer a clinical decision support system for multi-omic biomarker therapy planning using the precision radiogenomic classifier. In conclusion, we expect the integration of artificial intelligence tools and miRNA profiling in the clinical setting to allow the "personalization" of treatment and management, as is essential in a precision medicine era.
[1]Aerts H.J., et al., Decoding tumour phenotype by noninvasive imaging using a quantitative radiomics approach, Nat Commun. 5 (2014) 4006
[2]Saha A., et al., A machine learning approach to radiogenomics of breast cancer: A study of 922 subjects and 529 DCE-MRI features, Br J Cancer. 119 (2018) 508-516
[3]Grimm L.J., et al., Computational approach to radiogenomics of breast cancer: Luminal A and luminal B molecular subtypes are associated with imaging features on routine breast MRI extracted using computer vision algorithms, J Magn Reson Imaging. 42 (2015) 902-907
[4]Tahmassebi A., et al., Impact of machine learning with multiparametric magnetic resonance imaging of the breast for early prediction of response to neoadjuvant chemotherapy and survival outcomes in breast cancer patients, Invest Radiol. 54 (2019) 110-117
[5]Cain E.H., et al., Multivariate machine learning models for prediction of pathologic response to neoadjuvant therapy in breast cancer using MRI features: A study using an independent validation set, Breast Canc Res Treat. 173 (2019) 455-463
[6]Ha R., et al., Prior to initiation of chemotherapy, can we predict breast tumor response? Deep learning convolutional neural networks approach using a breast MRI tumor dataset, J Digit Imaging. 32 (2019) 693-701
[7]Miele, E., et al., Downregulation of miR-326 and its host gene ß-arrestin1 induces pro-survival activity of E2F1 and promotes medulloblastoma growth. Molecular Oncology, 2021. 15(2): p.523¿542
[8]Ferretti, E., et al., Interrogating molecular data for medulloblastoma risk stratification. The Lancet Oncology, 2018. 19(12): p. 1548¿1549
[9]Chiacchiarini, M., et al., Role of tissue and circulating microRNAs and DNA as biomarkers in medullary thyroid cancer. Pharmacology and Therapeutics, 2021. 219: p.107708