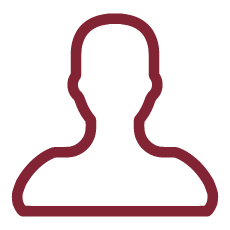
Radiomic is a methodology of advanced quantitative image analysis which uses complex mathematical algorithms to extract characteristic features from imaging data.
Hypothesis:
Radiomic analysis can predict outcomes following standard treatment in rectal cancer patients. We hypothesize that MR imaging¿based radiomics may add value to the current MR imaging assessment for evaluating patients with locally advanced rectal cancer after CRT, improving on qualitative assessment to differentiate patients with clinical partial response from those with cCR after CRT.
Aims:
1.To perform Radiomic evaluation of Patients affected by rectal cancer;
2.To correlate and find radiomic features linked to known prognostic factors;
3.To identify radiomic features able to aid in prediction of pathologic complete response (pCR) and local relapse.
Methods:
50 patients with locally advanced tumour stages II (cT3-4, N0, M0) and III (cT1-4, N+, M0) will be included in this non-randomized, prospective, single-center, trial. After the pre-treatment MRI, CRT will be administered according to international standard protocols. Between six and eight weeks after the treatment, a second post-treatment MRI will be acquired followed by total mesorectal excision (TME). During all MRI examination, tumor segmentation will be performed to extract radiomic features from T2, DWI and DCE. After the segmentation process, segmented images will be computed with Radiomic textural analysis method.
Expected results:
We expect that the radiomics analysis will show better classification performance compared with the qualitative assessment for diagnosing pCR in patients with locally advanced rectal cancer after
neoadjuvant therapy. This radiomic evaluation may become a potential imaging biomarker in the management of rectal cancer after neoadjuvant treatment.
Radiomics has emerged as a new research field with the aim of identifying quantitative medical image features associated with outcomes, building predictive models of outcomes, and thereby better understanding the underlying mechanisms of outcomes. With Radiomics, hundreds of quantitative image features from clinical images can be extracted using (semi)automatic software. The study of radiomics in rectal cancer patients is a new and emerging translational research topic. Radiomics in rectal cancer is frequently done to potentially improve diagnosis and characterization, mostly using Magnetic Resonance Imaging (MRI).
Texture Analysis (TA), recently introduced in the study of oncological images as a new Radiomic biomarker, consists in various mathematical methods that can increase the information obtainable from medical images, by quantifying a set of features that reflect the structure and the organization of the tissues within the ROI (Region of Interest) that delimits the tumor region.
Early diagnosis and accurate staging of rectal cancer are raising an essential role in the oncologic patients¿ management particularly in personalized treatment strategies.
Nowadays, magnetic resonance imaging (MRI) is considered the imaging modality of choice for loco-regional staging of rectal cancer. The validation of the technique for this purpose has been based on the ability to distinguish normal rectal wall from pathologic tissues on the basis of the high contrast resolution achievable on T2-weighted sequences. However, its role in the evaluation of response to therapy is challenging due to the difficulty in discriminating fibrotic to viable residual tissue after neoadjuvant CRT through morphologic approach with T2-weighted image.
Visual assessment has several limitations compared to quantitative measurements, such as inferior inter-reader agreement due to human eye error. Taking into account the weaknesses in visual process, there is the need to find new accurate quantitative semi- automatic/automatic diagnostics techniques. Recently, new MRI biomarkers, such as Texture analysis, were investigated. Texture analysis is a non-invasive method to evaluate tissue heterogeneity of tumor lesions. In literature, Texture parameters derived from T2-weighted images of rectal cancer have the potential role as imaging biomarkers of tumoral response to neoadjuvant CRT. Texture analysis evaluates the spatial variation of gray levels within an image, through mathematical equations that generate several parameters associated with the Texture of an image. It is possible to extract Texture parameters using statistical (first-order, second-order, and high-order), model-based or transform methods. In particular, Haralick¿s Texture analysis, known as the spatial gray-level dependence matrix method, let the study of second-order statistics of pixels at different spacings and angles of adjacent or nearest-neighbor pixels; this statistical method reflects the spatial and signal intensity interrelationships between adjacent in-plane voxels (e.g. contrast, homogeneity, second-order entropy and energy), useful for a quantitative analysis of the tissue corresponding to different histology observed for instance in fibrotic tissue instead of viable residual tumor.
An early prediction of patients who can respond to therapy or not should be advisable in future target and it will allow a considerable change in patients¿ treatment management.
Radiomics analysis of MRI images may potentially improve the discrimination between pathologic complete and pathologic partial response in patients with locally advanced rectal cancer after chemo- therapy¿radiation therapy.