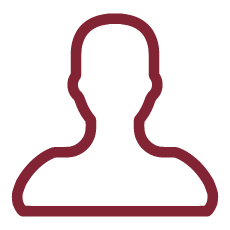
Radiomics, the application of mathematical algorithms to quantify the analysis of imaging data, has shown promising results in characterizing tumours (i.e., grading, aggressiveness) and in assessing response to treatment and outcome in oncologic Patients. Preliminary results are available for different cancer histology, including Non-Small Cell Lung Cancer (NSCLC). No relevant data are currently available in assessing response and outcome evaluation in patients affected by NSCLC and treated with anti PD1/PLD1 inhibitors.
Hypothesis:
The study hypothesis is that radiomic analysis can predict the response and outcomes in patients affected by Non-Small Cell Lung Cancer (NSCLC) and treated with anti PD1/PLD1 inhibitors.
Aims:
1.To perform Radiomic evaluation of Patients affected by lung cancer;
2.To correlate and find radiomic features linked to known prognostic factors;
3.To identify radiomic features able to aid in prediction of complete responder patients.
Methods:
50 patients with NSCLC will be included in this non-randomized, prospective, single-center, trial. After the pre-treatment CT, immunotherapy will be administered according to international standard protocols. After twelve weeks after the treatment, a second post-treatment CT will be acquired. During all CT examinations, tumour segmentation will be performed to extract radiomic features from non contrast and portal venous phase acquisitions. After the segmentation process, segmented images will be computed with Radiomic textural analysis method.
Expected results:
We expect that the radiomics analysis will show better classification performance compared with the qualitative assessment for diagnosing patients with NSCLC after immunotherapy. We also expect to identify radiomic features able to predict patient who will respond to immunotherapy and patients who will not. This radiomic evaluation may become a potential imaging biomarker in the management of lung cancer after treatment.
Radiomics has emerged as a new research field with the aim of identifying quantitative medical image features associated with outcomes, building predictive models of outcomes, and thereby better understanding the underlying mechanisms of outcomes, in particular, but not exclusively, in oncologic Patients. With Radiomics, hundreds of quantitative image features from clinical images can be extracted using (semi)automatic software. The study of radiomics in lung cancer patients is a new and emerging translational research topic. Radiomics in lung cancer is frequently done to potentially improve diagnosis and characterization, mostly using CT.
Texture Analysis (TA), recently introduced in the study of oncological images as a new biomarker, consists in various mathematical methods that can increase the information obtainable from medical images, by quantifying a set of features that reflect the structure and the organization of the tissues within the ROI (Region of Interest) that delimits the tumor region.
Early diagnosis and accurate staging of NSCLC are raising an essential role in the oncologic patients¿ management particularly in personalized treatment strategies and immunotherapy response evaluation.
Nowadays, CT is considered the imaging modality of choice for loco-regional staging of lung cancer.
Visual assessment has several limitations compared to quantitative measurements, such as inferior inter-reader agreement due to human eye error. In addition, tumor biopsy does not really reflect the entire tumor heterogeneity; to overcome these issues, a multiparametric approach have been proposed with improved results but not already optimal to assure a personalized treatment to patients.
Taking into account the weaknesses in visual process, there is the need to find new accurate quantitative semi- automatic/automatic diagnostics techniques. Recently, new CT biomarkers, such as Texture analysis, were investigated. Texture analysis is a non-invasive method to evaluate tissue heterogeneity of tumor lesions. In literature, Texture parameters derived from CT and PET/CT images of lung cancer have the potential role as imaging biomarkers of tumor response to immunotherapy. Texture analysis evaluates the spatial variation of gray levels within an image, through mathematical equations that generate several parameters associated with the Texture of an image. It is possible to extract Texture parameters using statistical (first-order, second-order, and high-order), model-based or transform methods. In particular, Haralick¿s Texture analysis, known as the spatial gray-level dependence matrix method, let the study of second-order statistics of pixels at different spacings and angles of adjacent or nearest-neighbor pixels. This statistical method reflects the spatial and signal intensity interrelationships between adjacent in-plane voxels (e.g. contrast, homogeneity, second-order entropy and energy), useful for a quantitative analysis of the tumor differentiating for instance pseudo-progression from confirmed progression.
An early prediction of patients who can respond to immunotherapy or not should be advisable in future target and it will allow a considerable change in patients¿ treatment management. Radiomics analysis of CT lung cancer images may potentially improve the discrimination between responder and non-responder to immunotherapy in patients affected by NSCLC.