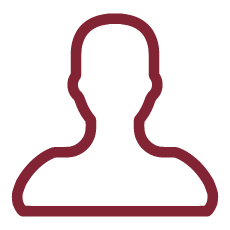
Among the available high-risk settings, aviation is usually referred to be a pioneering sector for higher safety management standards. The ways in which aviation service providers monitor safety performance is strongly influenced by international regulations, standards and agreements, although each State may also add its own local requirements. Particularly in the case of more mature organizations, the regulatory safety performance obligations are merely the tip of the iceberg in the undertaken safety performance activities.
In modern settings, the usage of Business Intelligence (BI) and Machine Learning (ML) solutions might become reference tools under the continuous chasing of strategies to foster organizations¿ safety intelligence capacities in such a high-risk system. The purpose of this project is to develop an integrated data-driven framework for self-service BI and ML on safety data for aviation systems. The proposed framework focuses on the development process of a BI architecture to extract meaningful knowledge from multiple data sources. Then, it progresses discussing how a ML solution may support to gain a deeper understanding of system¿s performance and to delineate specific safety recommendations. The analysis integrates a data-driven analysis into a functional representation of the high-risk world.
The contribution of this research is expected to define a democratized approach to BI and ML for high-risk settings, supporting a safer and more sustainable development of future systems.
The usage of aviation safety data represents the central data source for the proposed BI functional model in this explorative contribution for ATM safety performance. The proposed model encompasses multiple data sources referred to operational data. The BI-ML framework is intended to collect data coming from a variety of sources, following a novel multi-dimensional perspective. For example, investigation data in TOKAI may be linked to other data sources such as safety recommendations, or system changes. These items are helpful to assess the system functioning according to a more systemic perspective: not stopping the analysis at the individual investigation but including operational conditions (system changes) and what comes from its development (recommendations), and moreover attempting to make sense of the whole, rather than individual components.
A set of data sources may help adding further information (e.g.) a taxonomy of occurrences to ensure standardized labels for each safety event, latitude and longitude of relevant locations and hotspots (airport, radar, weather station, etc.), data on the structure of the airspace and its possibly dynamic classification/categorization, and basic information on airlines to be linked to airline and flight codes (e.g. aircraft call sign). Furthermore, the BI-ML framework will encompass the self-extraction and incorporation of data-driven taxonomies from investigation data via Machine Learning solutions, (e.g.) standardized EU Descriptive Factors or the event Explanatory Factors (taxonomy developed and currently available from EUROCONTROL). Both these factors can be used by investigators to describe the main features of the occurrences, or to provide functional explanation of main contributing or mitigating factors, in line with modern safety science research grounded in Safety-II (EUROCONTROL, 2015). In the BI-ML framework, these taxonomies will be built dynamically, according to a set of pre-defined rules, depending on the specific reported factor. This result will offer a novel perspective on the usage of such factors, currently using in terms of their frequencies.
The data model, enriched by the BI-ML framework and linked to functional variables through the MARIA model will offer the unprecedent ability to define exploratory safety indicators at systemic level.
For example, one of these KPI (Key Performance Indicators) may focus on the identification of anomalous behaviors in the number of percentage of safety recommendations coming from investigations that lead to actually approved safety proposals (anomaly detection). This indicator could be correlated to another KPI related to the quality level to identify groups of common investigations (clustering algorithm) to identify common issues that would not be shown on an individual assessment. In broader terms, from the combination of these types of KPIs arise the opportunities for proactive organizational learning, fostering discussion and information sharing within the organization, and also in relation with other stakeholders.
On a BI-centred perspective, the simple count of events (possibly averaged on traffic) can be related to the usage of specific factors (descriptive or explanatory), in order to find the most frequent causes. This individually frequentist analysis can be conducted also in a more systemic perspective, i.e. exploring the usage of joint factors via ML techniques based on supervised or unsupervised clustering (Hirose and Sawaragi, 2020). This additional KPI allows defining critical functional areas based on the joint reporting of multiple contributory factors, i.e. the more two factors are reported together the more robust is their link and effect on operations.
Additionally, investigation data can be possibly linked to weather information, in relation to both reporting data during the investigation and information coming from METAR (Weather Radar) and TAF (Terminal Aerodrome Forecast). This KPI might be used to check the consistence of weather forecats if compared to radar reporting and see if there is any link with some specific types of events, and unveil correlation from historic fit (defining key influencers, and anomalies).
EUROCONTROL, 2015. Eurocontrol¿s Learning Cards [WWW Document]. Edited by Shorrock, S. URL http://ansp1.azurewebsites.net/DownloadSafetyLearningCards (accessed 9.23.2020).
Hirose, T., Sawaragi, T., 2020. Extended FRAM model based on cellular automaton to clarify complexity of socio-technical systems and improve their safety. Saf. Sci. 123. https://doi.org/10.1016/j.ssci.2019.104556