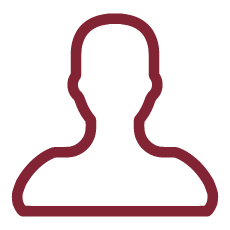
Artificial Intelligence (AI) and specifically Deep Neural Networks made incredible advances in the past few years transforming the way researcher analyse the ever increasing amount of data that today we are able to collect and store. This AI revolution is deeply impacting our society, from preventing disease, to building smart cities, to revolutionising analytics, up to art-producing neural networks that rival human creativity, are just few examples of applications based on Neural Networks that are routinely used today.
As the panorama of AI applications expands the deep neural networks are getting increasingly complex and demand unprecedented levels of computer power. In such scenario to be able to contribute and compete worldwide to the design, development and applications of the next generation of Deep Learning algorithms, it is crucial to have access to dedicated systems able to accelerate new AI model types that were previously untrainable, systems that outpace traditional data center architectures.
In this context we propose to buy a state of the art computing system for advanced Artificial Intelligence computation to be hosted in the computing center of the Department of Physics at Sapienza University of Rome and to use the system to develop innovative methods in the field of theoretical and applied machine learning, and to train students at undergraduate and graduate levels in physics, data science, mathematics, neuro science, medical diagnostic and imaging, and architectural design.
The proposed system will place Sapienza among the best universities worldwide for research and training in AI and Machine Learning.
As described in the previous section, the driving idea behind this project is choosing a certain number of lines of research in AI and Machine Learning for which new advancements would:
a) Be the result of really innovative Research and Development;
b) Bring useful and interesting results in the specific research sector where the research is developed;
c) Offer clear prospects for real impact in other scientific domains;
As an example of the concept let's discuss the line of research related to the development and application of innovative Artificial Intelligence methods in experimental particle physics. The computing power, flexibility and accuracy of the proposed GPU based low-precision and ultra-deep neural network architectures optimised for extremely sparse data, would provide a perfect solution for the next generation of experiments at the CERN Large Hadron Collider, and in general for any high rate/high complexity/high background real time filter system to be deployed in particle physics experiments. If successful the new methods will open the access to large samples of rare and complex to reconstruct signatures, such the ones from long-lived neutral particles predicted by dark-sector models, a very interesting class of new physics models that provides an explanation for the Dark Matter problem, one of the most compelling open question in fundamental physics today.
More efficient applications of large deep neural networks has also strong relevance in other fields of science and technology: can be applicable in all research areas which requires analyzing large amounts of data in real-time environments with a large potential for application in bio-medical imaging and radiation/particle-based therapies (for example in developing and deploy real-time systems for quality assurance in tomotherapy or medical image analysis).
Being able to develop more stable and robust techniques based on Generative Adversarial Networks will substantially improve the ability of the big particle physics experiments to produce large samples of simulated data, expanding the discovery potential for new physics effects.
A fast yet reasonably accurate simulation technique for electromagnetic and hadronic showers would be of great interest also in field outside the High Energy Physics. In medical physics Monte Carlo techniques are largely used for dosimetry calculation, for radiotherapy ( with photons, ions, protons), PET treatment, and also for calculating the shielding needed for the radiotherapy facilities. While most of these applications require a rather precise simulation, all of them would benefit from the use of faster techniques.
Another striking example of possible progress beyond state of the art in Deep Learning it is provided by the researches proposed in this project related to the analysis and description of the behaviour of Deep Neural Networks with the methods of Statistical Physics and Complexity Theory.
Unlike a researcher, neural networks can't explain their thinking: the computations that lead to an outcome are hidden. This motivated an effort to open up the black box of deep neural networks, building confidence in the insights that they yield. Understanding how and way these algorithms work so successfully is likely to become more and more urgent as AI's role in science, technology and society expands.
To be able to provide insights in a field of study like this, still completely unexplored , has the potential to provide important breakthroughs for the development of the next generation of Artificial Intteligence systems.