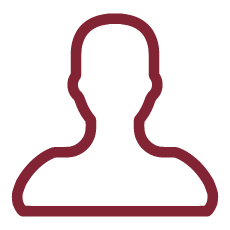
A strategic issue in circular economy consists in supporting new technologies, products and services related to Second Life Batteries, consisting in re-using end-of-life automotive Battery Energy Storage Systems (BESSs) in stationary applications. A key aspect for ensuring an effective reemployment of aged BESS will be the development of accurate and flexible models of electrochemical cells. Indeed, the availability of these models will be useful for performing accurate determination of the actual State of Health (SoH) of the cells, as well as accurate estimation of their State of Charge (SoC). Therefore, this project aims at developing suitable models of electrochemical cells characterized by a very flexible and accurate system identification procedure. During the project, the model will be used for performing SoC and SoH estimations, together with optimal balancing of the BESS. Moreover, it is expected to develop a Battery Management System prototype for evaluating the performances of the developed algorithms in a realistic experimental setup.
The main novelty and industrial benefit of the proposed modeling approach consists in the flexibility of the related system identification procedure. Indeed, thanks to the learning capability of NNs it will be possible to use data collected during the regular activity of the BESS in order to train specific models for each cell composing the battery pack. Conversely, conventional system identification procedures require to disassemble the BESS, and to perform specific, rigid and time consuming tests for completing the same task. Moreover, often expensive measurement equipment, such as programmable battery cycler and temperature chambers, are required. It is clear that those procedures are very unpractical for second life BESSs, because of the necessity of characterizing every dismissed cell for retrieving information about their residual usability.
Beside the advantage of a cheaper and less time consuming system identification procedure, the use of data collected during the regular activity of the cell has two further benefits. First, cell models will be tailored on data that matches the realistic behavior of the cell on the application in which it is used. Conversely, conventional system identification procedures will tailor the model on data that often is totally uncorrelated to the application of the BESS. Second, the data used for training the model comes from the same measurement equipment of the application in which the BESS is installed. This condition will allow to avoid any possible unexpected error due to different specifications of the sensing devices.
Regarding the BMS algorithms, the development of accurate and flexible models of electrochemical cells is beneficial first of all for performing likewise accurate SoC estimations. Indeed, the most promising methods proposed in the literature are the state-observer techniques [1], which effectiveness is strongly dependent on the availability of cell models. Moreover, an accurate determination of the SoC of every cell will make possible the development of innovative and more effective balancing algorithms that works by leveling the SoC of the cells instead of their voltages [2]. Finally, the proposed modeling technique will be beneficial for SoH estimation. Indeed, the flexible system identification procedure allows to perform a periodic update of the model parameters avoiding to put out of service the BESS. This way, it is possible to track the changes of the cell behavior due to the aging process. Moreover, the hybridization between equivalent circuit and NNs characterizing the proposed model allows to easily retrieve information about the internal resistance of the cell, that is commonly used for performing SoH estimation [3].
[1] M. Luzi, M. Paschero, A. Rossini, A. Rizzi, and F. M. Frattale Mascioli, ¿Comparison between two nonlinear Kalman filters for reliable SoC estimation on a prototypal BMS,¿ IECON 2016, Oct 2016, pp. 5501-5506.
[2] M. Luzi, M. Paschero, A. Rizzi, and F. M. Frattale Mascioli, ¿A binary PSO approach for real time optimal balancing of electrochemical cells,¿ in 2018 IEEE WCCI 2018.
[3] M. Berecibar, I. Gandiaga, I. Villarreal, N. Omar, J. Van Mierlo, P. Van den Bossche, ¿Critical review of state of health estimation methods of Li-ion batteries for real applications¿, Renewable and Sustainable Energy Reviews, Vol. 56, pp. 572-587, 2016.