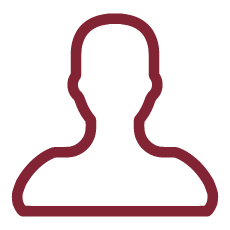
The Selective Laser Melting (SLM) is a promising Additive Manufacturing technology able to fabricate full density and high-performance 3D complex objects. However, the current attainable surface roughness is a limitation for industrial employment since it often requires secondary finishing operation which gives technological constraints and additional costs. Notwithstanding the efforts paid by the researchers, the surface improvement of those materials characterized by high reflectivity and conductivity (e.g. Aluminum alloys) are yet marginal. Remelting techniques have been proposed but they are limited to flat surfaces. In this project a new idea in the SLM processing is conceived: the aim is to provide an innovative way to promote a skin remelting stage which is applied to any inclined zones in a customizable way.
The remelting process is investigated and modeled to explore the obtainable roughness improvements together with possible underskin defects. The employment of the machine learning allows to classify and predict a complex set of surface quality outcomes (e.g. roughness parameters, defects, cracks and microstructure) as a function of several processing parameters. The feasibility of the methodology is strictly linked to the 3D data management. The method implementation is divided into two main stages: a new way to define 3D surface requirements and a designed for the purpose geometry segmentation which allows the material definition; a huge material database to be used directly in the SLM machine with an automatic coding. The collection of the abovementioned tools will allow to enrich the at present SLM technology with a locally customizable laser finishing operation in the same single-step process. Many other goals will be reach: drastic reduction of attainable roughness, assigning of a desired roughness to specific zones, fabrication of homogeneous quality parts, optimization of production time vs part quality vs part defects.
The novelty of this project can be categorized at three levels:
- Physical process: an in-depth analysis of the SLM remelting allows determining a big set of processing parameters combinations all over the local stratification angle i.e. on complex surfaces. In fact, current literature works on remelting mainly address their efforts on horizontal surfaces and rarely on vertical ones. Moreover, the literature shows a marked improvement for AlSi10Mg: Fig. 4 on the previous section shows the results of our two-years preliminary trials where the proposed LCSR improved the roughness by 93%.
- New metallurgical analysis criteria designed for SLMed parts for defect classification, detection and quantification. Neural network approach is employed for classification supported by digital image processing techniques. Together the roughness parameters data, the artificial neural networks provide comprehensive relationships between processing parameters, geometry, surface requirements and achievable surface quality and material properties.
- A novel dataset of materials, in place of the commercial sets, to be used in SLM of AlSi10Mg. The preparatory phase empowers a machine with a range of possibilities to build parts locally different in a pre-designed way.
- Innovative method to manage the part quality requirements on 3D model and new solution to the geometrical feature separation and assembly.
- Novel straightforward method to implement the methodology in the current machine build software to fabricate 3D geometry locally characterized by different surface quality and material properties.
The project proposes a novel paradigm because, for the first time, the part is not a single entity but is composed by separated features that are treated in different ways; however, the fabrication remains a single-step process.
This methodology can empower the possibility to fabricate prototypes satisfying the most or all the desired surfaces quality just at the end of the SLM fabrication: it finds direct application, for example, in aerospace or biomedical field where the surfaces are markedly affecting the functionality and their features are obtained, at present, by expensive and long secondary technologies which restrict the design freedom. Until now, the difficulty the utilizers find at their first approach to SLM has been plaguing the will to use this technology especially when they face the world of traditional and non-traditional conditioning operations necessary to obtain a working component. This is far from the process route simplicity of the digital manufacturing they suppose.
The aim of the project is riddled with a lot of challenging objectives. Notwithstanding the last research years of the proponents allowed to achieve several findings and now the elements are mature enough to develop this complex scope. The figures 4-7 and 10 shows results coming from procedures, experimentations, algorithms developed to demonstrate the feasibility of the physical, mathematical, computational and technical systems. We believe that the acquired skills and knowledges in several years can be coupled in this project.
The opportunity of this project, of course, include the technological transfer to the industry that will benefit from the implementation of this method in industrial production not limited to prototypes fabrication but especially for small and medium batched where the Industry 4.0 concepts strongly require the modeling of the SLM in order to maximize the flexibility and the productivity in a computer integrated and managed manufacturing.