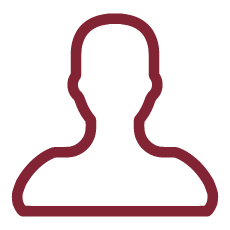
In this research, we develop a novel application of the synthetic control method proposed by Abadie et al. (2010) to evaluate the causal impact of carbon pricing on aviation supply. We exploit the policy change in the European Union Emission Trading System (EU ETS), the first large greenhouse gas emissions trading scheme in the world. Aviation has been included in the ETS in 2012, thus making carbon dioxide (CO2) emissions a new input cost for the air travel industry.
In order to evaluate the effect of the EU ETS on aviation supply, we compare the evolution of the outcome between the routes affected by the EU ETS (i.e., the treatment group) and the unaffected routes (i.e., the control group). The selected aggregate outcome is expressed in terms of airline output (i.e., number of seats supplied at the airline-route level). We distinguish between low-cost and full service airlines, short and long-haul routes, routes towards (or from) hub airports vs non hub airports and, because the number of seats is a combination of flight frequency and aircraft size, we also examine the effect on average aircraft size.
In our evaluation of the EU ETS effect, synthetic control methods are particularly relevant, as each synthetic control provides the counterfactual outcome trajectory that the corresponding affected route would have experienced in the absence of the EU ETS policy. In addition, if the approximation given by synthetic controls is poor, the discrepancy (i.e., the lack-of-fit) can be computed and visualized in the time period prior to the EU ETS intervention. That is, if the fit is poor, we would know that synthetic controls are not appropriate to use in our EU ETS study. Abadie, A., Diamond, A., and Hainmueller, J. (2010). Synthetic control methods for comparative case studies: Estimating the effect of california¿s tobacco control program. Journal of the American Statistical Association, 105(490):493¿505.
The contribution of our project is twofold.
First, some earlier studies have identified causal impacts of carbon pricing in a specific country and at the firm level with a focus on the propensity towards low-carbon investments (see the literature section). Conversely, we measure a whole market¿s reaction to carbon pricing as we investigate causal impacts of carbon pricing in the aviation sector whose business is not limited to just one particular administrative area. To the best of our knowledge, our study is the first empirical attempt to evaluate (at the market level) the causal impact of carbon pricing on aviation supply exploiting a policy change in the EU ETS.
Second, we provide a novel application of the synthetic control method by offering two major tweaks to the methodological framework proposed by Abadie et al., (2010).
On the one hand, we offer alternative ways to provide theoretical justification for constructing the weights. Note that synthetic control is a weighted average of the available control units. By doing so, the synthetic control method makes explicit: (1) the relative contribution of each control unit to the counterfactual of interest; and (2) the similarities (or lack thereof) between the unit affected by the event or intervention of interest and the synthetic control, in terms of preintervention outcomes and other predictors of postintervention outcomes. In the construction of the weight vector, Abadie et al., (2010) proved asymptotic consistency under the assumption that the number of pre-treatment periods approaches to infinity. The asymptotic result is not very relevant to our application, as our data only cover 5 pre-treatment periods (i.e., 5 years pre-ETS). We then provide a novel theoretical justification for constructing the weights based on the unbiasedness argument. Our result is relevant to applications of the synthetic control method characterized by a relatively small number of pre-treatment periods.
On the other hand, if we apply the existing methodology in Abadie et al., (2010) in an off-the-shelf fashion to our framework, then the nested minimization needs to be done 52,158 times and each minimization involves the search of a weight vector of length 19,950. This approach is theoretically sound but computationally infeasible. In fact, even with parallel computing on a computer cluster with 100 CPUs, the run time for this computational undertaking is estimated to be six years, making the implementation practically impossible. We then propose a stochastic approximation scheme, which, in the given framework, has allowed us to complete all the numerical optimizations in two months.