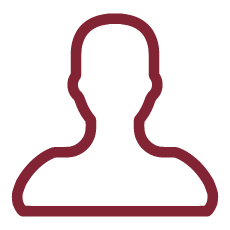
Over the past seventy years, much research on automatic music generation has been conducted. From melody creation to chord progression, computer scientists, along with music experts, have applied increasingly sophisticated computational methods to the various tasks that make up the creative process.
Quite recenty, the impressive advances in machine learning - as well as the sharp decrease in computational costs - have led to a leap forward in automatic music production. After years of limited results, at the beginning of 2020 OpenAI researchers released a novel deep learning architecture that is able to effectively generate new songs. Trained on more than one million songs, OpenAI Jukebox has successfully addressed many tasks that the MIR community has been chasing for decades.
Following this new promising path, in this project we want to design and train a transformer-based maching learning algorithm that would be able to produce a convincing sound landscape around an acappella song given as input. To the best of our knowledge, we are the first to address this specific generation task in the music field. Using the mel-spectrogram representation of a large corpus of songs, we will teach our model to complete spectrograms where only partial information - i.e. the vocals - is available.
To the best of our knowledge, we are the first to address the problem of automatic music arrangment. Our research would allow to fill an important gap in our understaing of deep musical structures. Indeed, training our model on a large corpus of modern songs, ideally we would be able to discover frequency relations among all the salient parts of a piece of music and exploit them to generate a full song given an unseen sung melody.
Our research question is interesting from both an applied machine learning point of view and from a more theoretical standpoint. And this because the underlying problem concerns the estimation of the marginal distributions of an highly multi-dimensional probabilistic model.