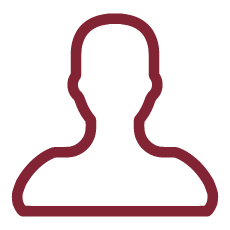
The world is rapidly approaching 10 billion people. Growing enough healthy food under the added stress of climate change
represents a significant challenge.
Agriculture technological development plays a major role in addressing the above challenge because especially in developing countries, people health, population growth and the same country economic survival, are almost exclusively based on agriculture production.
This brings up the need to foster the utilization of the most updated systems for pest and disease surveillance.
Nevertheless, even the most updated systems for crop surveillance are reactive where observations by farmers, survey teams or extension workers provide presence/absence maps that are intended to lead to responses. Given the infectious nature of pests and diseases this approach allows the pest/disease agent to expand, leading to epidemic outbreaks (e.g. rusts) or establishment where eradication is no longer considered feasible (fall armyworm in Africa). As such, the collected surveillance data leads only to a form of triage.
We propose a different approach which is a predictive platform that determines where diseases and pests are likely to occur using historical data, multi-scale weather models, remote sensing and Artificial Intelligence (AI) enabled ground observations.
We envision the use of a new pioneering network of flying monitoring drones to detect ongoing diseases and to predict
their development, and study the speed and direction of their propagation while the epidemic outbreak is still in progress and can be kept confined and under control.
Drones and ground devices can support each other to perform disease recognition at the desired level of fidelity, and forecast the direction and speed of a disease propagation.
Our proposed tools offer the prospect of prediction or early discovery of a spreading disease that will allow informed decision making to keep diseases grographically confined and eventually defeat them.
Our proposal is innovative with respect to the current state of the art in many aspects.
In fact, previous proposals dealing with pest and disease monitoring rely on a reactive approach based on the only observation of anomalies on the field of interest.
Our proposal innovates with respect to these works in that it exploits results from epidemiology to realize prediciton tools coupled with the study of weather patterns and other environmental conditions that are known to promote pest and disease spread among crops.
By means of these studies we think we can shift from reactive to proactive approaches. Our idea is that the current system presents an opportunity for the application of algorithmic rules to forecast and determine risk.
As a second innovative aspect of our research we underline that current disease monitoring systems for agriculture rely on single monitoring devices. By contrast we propose an integrated system formed by cooperating aerial drones, working jointly with ground based sensors and mobile cellular phones (crowdsensing) to supply a thorough observation of the ongoing phenomena.
We envision the realization of an autonomously adaptive monitoring system provided by a squad of UAVs, combined with an intelligent system capable of recognizing images of plants and related diseases with autonomously tuned fidelity. A self-coordinating squad of autonomous drones will sample a region, and match the measurements with information related to environmental factors, such as weather forecast, to detect diseases and predict the speed and direction of their propagation while it is still in progress. Upon detection of a propagating disease, our system will trigger prompt intervention to perform deeper inspection (as in Fig. 2) and to enable actions such as protecting the smallholder farms not yet affected by the disease, treating the affected plants when possible, and enabling protection policies to stop or mitigate the disease propagation, such as the creation of "firewall" lines and the adoption of pest control chemicals or medicines.
While the use of single, remotely controlled, drone for precision agriculture is currently a hot research topic, the current project aims at
advancing the state of the art by integrating several key ingredients:
1. the use of a squad of multiple autonomous and cooperative drones. This includes the study of special purpose communication protocols for device path planning and cooperation, as well as routing schemes.
2. the use of machine intelligence systems for the recognition of plants and related disease from cameras and processors located
onboard the drones.
3. the integration of online available information on the environment, for prediction of future environmental conditions and factors (e.g. weather) that can potentially cause or foster a disease propagation.
4. the use of statistical sampling, the study of geographical correlation and spline interpolation of measurements to determine the
frontline of a spreading disease and its direction.
5. geographical scalability, which is achieved by trading off a triangle of settings including accuracy of detection, number of drones, and time to complete the monitoring tasks.
Joint autonomous path planning and dynamic task assignment on a squad of drones is something very new and unexplored so far. The use of a machine intelligence system, with tunable fidelity, for recognition of plant diseases is also at a preliminary stage, and most of the work has been done with same height imaging, while the use of drone based imaging is still a challenge for the specific angle of sight which requires adapting existing images archives.
The results of the project are of interest to many other application scenarios requiring situation awareness and preparedness. Monitoring systems are widely recognized to be an invaluable tool whenever surveillance, intrusion or hazard detection are required to ensure prevention, prediction and protection against critical events such as natural disasters or security threats.
The project focuses on the important application of crops in fields and ecosystems monitoring, with a large impact on food security, human safety, and global well being planet-wide. Additionally, the proposed system has the responsiveness, the robustness and the autonomous intelligence that make it suitable as well for other important applications such as disaster monitoring and homeland security.
Such an approach will immediately provide broader impacts. Hence, besides the innovation in the realization of autonomous squad of drones for monitoring systems, the project will provide important innovations in the field of food security, as we will have a smart autonomous system collecting millions of data points on crop type and disease in real work farms. Our monitoring system, in the hands of food security agencies and governments, will produce a considerable economic shift in low-income countries.