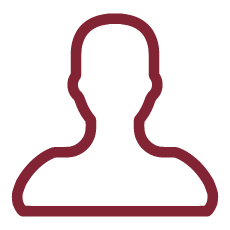
Handling populations' heterogeneity is a common issue in capture-recapture problems. To correctly estimate a target population's size, the possibility of heterogeneity, i.e. that capture probabilities vary among individuals, must be considered. Such difference in capture probabilities might be due to different "weights" the groups within the target populations have in the various capture occasions. Noncentral Hypergeometric (NH) distributions arise naturally in situations where units in the population that are sampled without replacement have different probabilities of being drawn. Such distributions have been underemployed in the statistical literature mainly because of the computational complexity given by their densities. Nevertheless, modern computational tools allow for the exploitation of such distributions, that are easily applicable in a variety of contexts. This project's aim is to give Fisher's NH distribution a new vest, using it in an official statistics context.
This project fits perfectly into the most recent research interests in the official statistics field. Population's size estimation via integration of multiple sources is a key issue for National Statistics Institutes, a hot topic they are expected to invest on for the next years. In this framework, the problem of dealing with heterogeneity in capture-recapture experiments is crucial. As underlined in (Johndrow et al. 2016), "the presence of capture heterogeneity is equivalent to bias in the sampling process"; although this statement might be seen as an explicit encouragement to the use of Noncentral Hypergeometric distributions, such models have not been used in this context yet. This class of distributions is still underemployed in the statistical literature, although the number of its potential applications is high. In fact, the little attention literature had paid to the NH distributions is certainly not due to their limits, but rather due to their complexity. However, modern technological tools and computational capabilities can now allow us to exploit such distributions in depth. This project brings together the need to make advances in the population's size estimation field and the possibility to explore an undervalued but powerful probability distribution, which potentially has many applications.