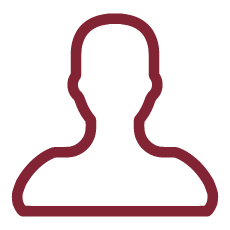
A fundamental milestone for quantum technology is represented by the capability of reaching the regime of quantum advantage, namely the scenario where a quantum device is able of solving a specific problem faster than any classical system. Boson Sampling, a classically-hard computational task, represents one of the most promising approach to reach such quantum advantage regime in a photonic platform. Besides the current advances in the technology, enabling the implementation of progressively larger photonic systems, a fundamental aspect can be found in the certification problem. More specifically, when reaching the quantum advantage regime where a classical system will not be able to solve the task, it is necessary to exploit suitable methodologies to certify the output of the corresponding quantum computation. In the Boson Sampling case, it is necessary to verify that the quantum device solving the task is sampling from the correct distribution, and is not affected by physically-motivated sources of noise. In this context, partial photon distinguishability is one of the most significant sources of imperfections, that can spoil the complexity of the classical computation if the noise level is above a given threshold.
In this project, we aim at developing and testing experimentally in an integrated platform validation tests capable of addressing certification against partial photon distinguishability. In particular, we will investigate the adoption of Machine learning protocols for such task, being the latter algorithms particularly suitable to deal with large data sets and to find hidden patterns. The obtained results are expected to increase the current state-of-art capability of verifying Boson Sampling computation, and are expected to be of significance for certification of other quantum computation and simulation platforms.
The aim of this project is to develop and test experimentally a validation test, based on Machine learning techniques, capable to address partial distinguishability in Boson Sampling experiments. Certification of quantum devices solving such problem is a fundamental task, in particular when dealing with a progressive increase of the system size (number of photons and modes) towards reaching the regime of quantum advantage. Indeed, in the latter scenario trivial methods cannot be applied, since it is not possible to calculate the expected output distribution neither to acquire sufficient data samples for its experimental reconstruction.
In particular, it is necessary to be able to recognize the presence of the most common sources of experimental imperfections in the output of a Boson Sampling experiment, with particular emphasis to those errors that spoil the complexity of the calculation. While losses or circuit errors can be characterized in advance directly on the implemented device, partial photon distinguishability has to be characterized directly on the measured data to avoid changing the experimental apparatus. Given the capability of Machine Learning protocols to handle large data sets and find hidden pattern without requiring a priori information on the system, we will apply such class of algorithms for Boson Sampling certification.
The expected outcome of this project will be a novel class of validation tests for partial photon distinguishability, capable to operate directly on the collected data without requiring additional resources or different input transformations. This capability of working directly on the detected data will be a significant feature, since it will avoid changing the experimental system for the certification process and thus it will not add any loophole due to this modification.
The results expected from this project will represent a boost in the capability of verifying the output of Boson Sampling experiments. Indeed, given the very complexity of the problem, it is expected that certification of such class of sampling problem will require a complex toolbox of protocols and algorithms [3,5], while no single algorithm will be capable to perform full certification [1]. Within this toolbox, each protocol will address a specific source of error in the experimental apparatus, thus providing a sequence of tests capable to lead to a progressively more refined and stringent certification of the quantum device. This will allow to progressively exclude all main sources of physically-motivated error models, thus increasing the confidence that the quantum device is working properly.
Furthermore, the application of Machine learning for Boson Sampling experiments will also be a demonstration of the potential application of this class of algorithms in validation tasks for other platforms. Indeed, certification and benchmarking of quantum systems is an ubiquitous task, which is common to different quantum computation and simulation problems [5], as shown in the recent experimental showing quantum supremacy with superconducting qubits [42].
[42] F. Arute, et al., Nature 574, 505-510 (2019).