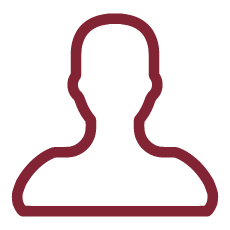
Student dropout prediction (SDP) is a specific problem in the multidisciplinary field of Learning Analytics (LA). It aims to analyze student withdrawal in distance learning environments by modeling the student behavior when interacting with e-learning platforms. Student dropout prediction should be treated with significant importance because, in the last decade, online courses have propelled a new era on education. Although online education systems have started in the mid 1990, little attention has been paid to the difficulties that these students experience during their studies. The recent diffusion of online courses (especially Massive Open Online Courses - MOOCs), with their enormous number of enrolled students - out of which only a fraction completes their studies successfully - has led to an increased interest on this problem. As a consequence, a growing number of online institutions have commenced to consider the adoption of automated strategies to help predicting their students' withdrawal decision.
In this project we propose to develop solutions based on data analytics and machine learning to support specific actions to favor the reduction of dropouts in distance degree courses. The possibility of analyzing data containing the "digital traces" of students enrolled in these courses (such as data on access to videotaped material, interaction on forums, questions to tutors, time spent listening to individual teaching modules, etc.), can support the development of advanced analytical models, which may favor the early identification of students in difficulty, and the development of customized actions.
As we already highlighted, drop-out prediction in on-line degree courses is particularly challenging, since student activities within an online learning platform are multiple and of a variegated nature: they are sequential (when student are engaged in activities related to single courses), parallel (since they usually attend more than one course) and interactive (on-line students, more than others, benefit from participating in course forums and student social networks). As a consequence, finding a model that suitably accounts for these multifaceted activities, as well as understanding their mutual influence in determining a student's performance, represents an open problem.
Although the recent availability of high-performing deep machine learning methods offers the possibility of integrating sequential and parallel data, coping with the full complexity of student modeling in distance learning environments is still to be explored, thus providing a wide opportunity of contributing to the academia with innovative ideas.
The innovative aspects of our project are both in the modeling and prediction strategy.
Similarly to some of the studies summarized previously, we propose to use sequence labelling to shape student data. With respect to the state of the art, the major foreseen innovations are the following:
1) Higher Complexity of the adopted student model: Most surveyed approaches consider student data from single MOOCs. Instead, we consider data concerning the entire set of e-tivieties of a degree course. This means that students' e-tivities should be modeled as a set of parallel and asynchronous sequences (since students may attend several courses in parallel, interact with other students and professors, etc.). Although adopting a more complex model is challenging, it may ultimately foster better predictions and interpretability of results.
2) Explainability of predictions: The current generation of Intelligent systems based on deep machine learning seem to be inscrutable. This is indeed a major obstacle, especially for applications whose main purpose is to support human decisions. Attentive models, that will be explored in our proposed study, are one of the possible solutions recently proposed in literature to help understandability and trust. However, state-of-the-art attentive networks do not readily apply to our student model. Adapting and understanding explainability mechanisms will be one of the expected results of this study.
Beneficiaries of the study:
Although it is expected that the results of the project, due to the methodologies adopted, can be largely generalized to all degree courses in distance learning, the proposed study will concern Unitelma degree students and Unitelma-Sapienza inter-university students. The database containing the "trajectories" of the students, currently being prepared and anonymized, concerns about 5,000 students for a duration of 5 years. Given the current literature, this will be the largest use case on the subject, since other studies are limited to individual MOOC courses (Massive Open Online Courses).
Potentially, however, the beneficiaries of the results, if positive, will be much larger. In Italy there are 11 certified telematic universities, and a growing number of state universities offer online courses, including, in addition to Sapienza, Turin and the Polytechnic of Milan. Abroad, the Online Studies sector portal has 4,429 digital programs on a global scale, of which 1100 in the USA alone (Sole24Ore, December 20, 2017).
In addition to the students actually enrolled in online courses, the potential of these courses must be taken into account, if it is possible to improve the quality of the service offered without significantly affecting costs. In Italy, more than 400 thousand non resident students (https://www.skuola.net/news/inchiesta/fuori-sede-universita-flussi-regio...) are distributed heterogeneously throughout the country. Most of them come from the regions of Southern Italy and head towards the large cities of the Center-North, with the greatest concentration in the Lazio region. On the other hand, to cope with the crisis and continue to study, 3 out of 4 students live with their family of origin and over half (50.1%) of the total choose to study in another city but continue to living with family members (http://scuola24.ilsole24ore.com/art/scuola/2015-11-04/studenti-lavorator...). Moreover, even if in the last 10 years, among all university students, in 2015 those working between the ages of 20 and 24 reach 64 thousand (http://www.ansa.it/sito/notizie/economia/2016/11/20/istat-sempre-meno-st...).
All this motivates the usefulness of degrees with online programs for commuting, off-site students and workers, since they offer flexibility of frequency during their studies.